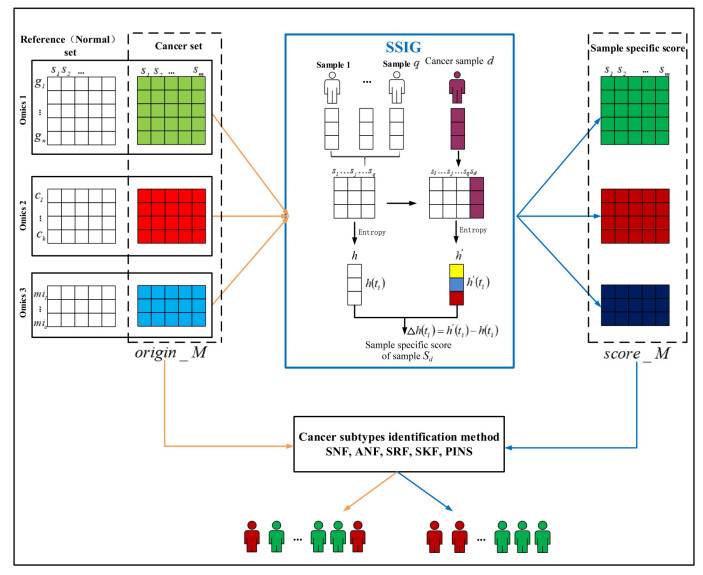
Citation: | ZHANG Yuanyuan, WANG Ziqi, WANG Shudong, KOU Chuanhua. SSIG: Single-Sample Information Gain Model for Integrating Multi-Omics Data to Identify Cancer Subtypes[J]. Chinese Journal of Electronics, 2021, 30(2): 303-312. DOI: 10.1049/cje.2021.01.011 |
Cancer is a heterogeneous disease that occurs in different parts of human body in different forms, forming different types of cancer. Even the same type of cancer will evolve into different subtypes due to various uncertain factors, which brings difficulties to the diagnosis and treatment of cancer[1]. Therefore, the key to personalized prognosis and treatment is to find out the mechanism of carcinogenesis through multi-omics datasets and data-driven way to identify cancer subtype.
With the development of high throughput technology, different omics data emerge, such as The cancer genome Atlas (TCGA)*, which is an open large-scale biological database, including more than 34 kinds of cancer and 15 kinds of biological data sets[2]. Due to the heterogeneity of cancer, a single omics data may not be sufficient to accurately detect subtype information. Therefore, in the past decade, many computational methods using multi-omics data to identify cancer subtypes have been proposed[3-5]. At present, the identification methods of cancer subtypes based on multi-omics data mainly include three categories: early integration, middle integration and late integration[6]. Among them, middle integration and late integration methods are the mainstream.
Similarity-based methods, such as Similarity network fusion (SNF), Affinity network fusion (ANF), Similarity regression fusion (SRF) and Similarity kernel fusion (SKF), belong to middle integration. SNF is proposed by Wang et al.[7] in 2014. It uses the information transmission theory to update the similarity network of different omics iteratively. In the iterative process, the sample pairs supported by strong edges in different omics sample similarity networks will be strengthened, while the weak edges caused by noise will be weakened until they disappear and converge to a single consistent sample similarity network. ANF is proposed by Ma et al.[8] in 2018. It avoids the need for multiple iterations to obtain the sample similarity network in SNF through constructing k-Nearest neighbor (k−NN) affinity network based on Gaussian kernel function. A comprehensive sample affinity network is obtained by combining the affinity networks of all omics and then spectral clustering is used to identify subtypes. SRF is proposed by Guo et al.[9] in 2018. It spreads other omics information in an omics network and uses rank transform to eliminate scale differences. Then, the transformed data is decomposed into several sorting factors, and different weights are given for different omics according to the importance degree. Finally, the multi-omics information fusion is carried out. SKF is proposed by Jiang et al.[10] in 2019. It constructs sample similarity kernels of multi-omics data, then builds sparse kernels and normalized kernels according to the information in similar kernels. In addition to their own kernel information as a supplement, the normalized kernel is updated iteratively to strengthen the samples with strong similarity in the kernel, while the samples with weak similarity will become 0. Finally, multiple similar kernels are fused into a sparse single kernel for cancer subtypes identification.
Late integration refers to the clustering of single omics separately and then the clustering results are fused. In 2017, Nguyen et al.[11] proposed a Perturbation clustering for data integration and disease subtyping (PINS) method. Different from the above methods, PINS integrate the clustering results by adding disturbance to the sample information. In order to check whether it can be clustered into smaller clusters and improve the robustness, Gaussian perturbation is added to the data. Finally, after the clustering effect converges, the cluster results of samples from multi-omics are highly consistent into one group. Therefore, it does not need to specify the number of clusters.
The methods of the above cancer subtypes have studied how to classify cancer subtypes and achieved fruitful results. Among them, SNF, ANF, SRF and SKF are based on the similarity fusion between samples, and PINS is based on the integration of common clustering patterns in different omics[4]. They use the multi-omics data in the population to find the common characteristics of the multi-omics data or clustering information in the population, and find the data consistency. However, the sample information fusion in the multi-omics may weaken the dominant influence of a single sample in a certain omics, ignoring the difference of cancer samples at the individual level[12]. Therefore, the identification of cancer subtypes based on single sample is a promising research topic.
In this paper, we proposed an SSIG model based on single sample. Sample specific score is given for each sample according to the change of information gain of single sample relative to reference data, it can quantify the difference between each sample and normal tissue, which can distinguish cancer patients and further classify cancer subtypes effectively. We used SSIG to obtain the sample specific score and applied them to five methods (SNF, ANF, SRF, SKF, PINS). The basic steps are as follow: 1) Select the appropriate multi-omics data (including normal data and cancer data) and survival data of samples; 2) We use SSIG model to obtain the sample specific scores of each omics after preprocessing multi-omics data; 3) The original multi-omics data and the sample specific score data are brought into the five cancer subtypes identification methods to obtain different cancer subtypes; 4) Clustering measure, survival analysis and heat map visualization are used to compare the performance of different methods. It is shown that sample specific score has better performance inavariety of evaluation indicators, and has obvious differentiation in the survival curve, which can better distinguish different subtypes of cancer.
In this section, we will introduce the SSIG model and its application in current methods for integrating multi-omics to identify cancer subtypes (see Fig. 1).
For any omics data, suppose that the omics has f features. The normal sample corresponding to a certain cancer is regarded as the reference samples. Therefore, for feature ti(i=1,…,f), the information entropy h(ti) under the reference samples is defined as:
h(ti)=−q∑Sj=1pSjlogpSj |
(1) |
where pSj=tSj/sum(tSj), tSj represents the value of feature ti under sample Sj,q is the total number of reference samples.
For one cancer sample Sd,tSd is the value of the sample d which is independent of the reference sample feature. The information entropy is recalculated by adding the sample to the reference samples as follows:
h′(ti)=−q+1∑Sj=1pSjlogpSj,j=1,…,q,d |
(2) |
The sample specificity score of cancer sample d under the feature ti is defined as Δh(ti)=h′(ti)−h(ti) which can be known as the sample specific information carried by the feature ti of the sample d.
Suppose that there are M omics data, and the m-th omic has fm(m=1,…,M) features. The values of all the features of all cancer samples under the m-th omic are called the original feature matrix, denoted by origin_M=(tij)fm×Nm, where Nm represents the number of cancer samples in the m-th omic. According to the SSIG model, the specificity score of each feature for each sample can be obtained. Therefore, we define the score matrix as score_M=(Δhij)fm×Nm. origin_M and score_M are used as the feature matrices of cancer samples respectively and applied to current prevalent five subtype identification methods[7-11]: SNF, ANF, SRF, SKF and PINS. For SNF, SKF, ANF and SRF four fusion methods, sample similarity networks are constructed based on sample feature matrix respectively, and then subtypes of cancer samples are identified by clustering; PINS method adds disturbance to the feature matrix of samples, and then selects robust clustering results, thus obtaining the subtypes of disease samples. We compare the performance of two different feature matrices origin_M and score_M for subtype identification among the five methods.
The following is a brief introduction to the basic principles and rules of the five methods for cancer subtypes:
SNF[7] First, a sample similarity network is constructed based sample feature matrix for each omics. Then, the iterative method is adopted to fuse the sample similarity network of different omics to obtain the fused sample similarity network. Finally, the spectral clustering algorithm is applied to the target fusion network to identify cancer subtypes.
ANF[8] ANF is a cancer subtype identification improved method based on SNF. It optimizes the method of obtaining target fusion network iteratively by SNF, proposes the method of obtaining sample similarity network by using k-NN Gaussian kernel method to fuse samples' multi-omics feature matrices. Finally, semi-supervised neural network is used to identify cancer subtypes.
SRF[9] SRF is proposed through considering the weight problem between different omics data and the similarity deviation of samples, used the generalized linear regression model to correct the similarity deviation between different omics samples, and carried out multi-omics data fusion under the premise of importance, so as to identify cancer subtypes.
SKF[10] SKF is a novel unsupervised multiple kernel fusion method. It continuously updates the normalized kernel through the sparse kernel in an iterative way. The purpose is to integrate three similarity kernels into one combined kernel. In the end, the information in the combined kernel is used for clustering.
PINS[11] PINS is a method of perturbation clustering. In this method, clustering is conducted in multi-omics respectively, and then noise disturbance is added into the data to select the most stable clustering result. Finally, the clustering information is integrated to achieve the goal of identification of cancer subtypes.
SNF, ANF, SKF and SRF are four middle integration methods to obtain the sample similarity network based on sample similarity fusion[13], and then conduct clustering analysis according to the obtained similarity network, so the user needs to specify the number of clusters[14]. In this paper, we set the number of clusters to 2, 3, 4, 5 and analyzed them respectively. PINS which is a late integration method can automatically select the optimal number of clusters. These five methods are used to evaluate the performance of SSIG model applied to the identification of cancer subtypes.
Considering the lack of a gold standard in the identification of cancer subtypes at present[14], we download two different types of cancer data, BRCA and KIRC, from the TCGA database as two types of real labels for the samples to test the performance of different methods for the identification of cancer subtypes.
For each cancer, we extracted three omics data, including gene expression of IlluminaHiSeq_RNASeqV2 platform, DNA methylation data of Illumina Infinium HumanMethylation27 platform and miRNA expression of IlluminaHiSeq_miRNASeq platform[15]. In addition, clinical survival data also be downloaded for each type of cancer. The original characteristics of the three omics data are shown in Table 1. For each cancer dataset, we matched the cancer samples in three different omics, considering the measure of specificity for each cancer sample. Then, due to measurement errors, there are many NA values in the omics data. We delete these features with NA values. And, since many genes have 0 expression values in the gene expression data, genes with 0 expression values provide little information and may mislead the results of certain methods[16]. Therefore, the gene whose expression value is 0 in more than 50% of the sample is removed. For genes whose expression value is 0 in less than 20% of samples, we adopt data that satisfy their normal distribution to fit these 0 values. The data fitted in this way will not change the entire data distribution and retain the characteristics of the original data to the greatest extent. At the same time, we ensure that the corresponding omics features in different cancers were matched. For each normal dataset, it is not necessary to match samples in three different omics. Because the normal data set is only used as a reference data set to calculate the information gain. But, we need to take the above method to remove 0/NA values and match the corresponding omics characteristics in different cancer types. The characteristics of the three omics data after processing are shown in Table 2.
Diseases | mRNA | DNA methylation | miRNA | |||
Genes | Samples | CpG sites | Samples | miRNAs | Samples | |
BRCA | 20531 | 1104(114) | 27579 | 318(27) | 2239 | 756(76) |
KIRC | 20531 | 534(72) | 27579 | 219(199) | 2049 | 241(70) |
Diseases | mRNA | DNA methylation | miRNA | |||
Genes | Samples | CpG sites | Samples | miRNAs | Samples | |
BRCA | 17487 | 130(114) | 20417 | 130(27) | 247 | 130(76) |
KIRC | 17487 | 63(72) | 20417 | 63(199) | 247 | 63(70) |
In order to compare the performance of SSIG model and the original data features applied to five methods of cancer subtype identification, three clustering evaluation measures, Silhouette coefficient (SC)[17], Normalized mutual information (NMI)[18], Adjusted rand index (ARI)[19], are used for comparison (Tables 3–5). SC which combines cohesion and separation two factors is an unsupervised clustering evaluation algorithm. Small intra-cluster distance and large inter-cluster distance represent reasonable clustering results. The value range of SC is [–1, 1]. The larger the SC is, the better the clustering result will be; NMI is a supervised clustering evaluation algorithm that measures distribution differences by calculating mutual information between clustering results and real results. The value range of NMI is [0, 1]. The larger the value is, the smaller the information between each other is, and the closer the clustering result is to the real result; ARI is a supervised clustering evaluation algorithm used to measure the consistency between subtype classification results and real results. ARI value range is from –1 to 1, higher value indicates better clustering performance.
Methods | k=2 | k=3 | k=4 | k=5 | ||||
Origin | Score | Origin | Score | Origin | Score | Origin | Score | |
SNF | 0.9817 | 0.9974 | 0.8120 | 0.6320 | 0.7278 | 0.6514 | 0.6563 | 0.4793 |
ANF | 0.8767 | 0.9820 | 0.8491 | 0.4722 | 0.5853 | 0.5456 | 0.5167 | 0.3260 |
SKF | 0.3698 | 0.4270 | 0.5502 | 0.4385 | 0.6034 | 0.4978 | 0.5826 | 0.5325 |
SRF | 0.0154 | 0.2258 | 0.0059 | 0.1265 | 0.0043 | 0.1028 | 0.0028 | 0.0476 |
Methods | k=2 | k=3 | k=4 | k=5 | ||||
Origin | Score | Origin | Score | Origin | Score | Origin | Score | |
SNF | 0.9547 | 0.9547 | 0.7480 | 0.7317 | 0.6494 | 0.6495 | 0.6074 | 0.6067 |
ANF | 0.9547 | 0.9547 | 0.7874 | 0.7697 | 0.6817 | 0.6826 | 0.6381 | 0.6349 |
SKF | 0.9547 | 0.9547 | 0.7445 | 0.7462 | 0.6489 | 0.6808 | 0.6054 | 0.6052 |
SRF | 0.9547 | 0.9547 | 0.7194 | 0.7462 | 0.5883 | 0.6531 | 0.5302 | 0.4528 |
PINS | − | 0.9547 | 0.8594 | − | − | − | − | − |
Methods | k=2 | k=3 | k=4 | k=5 | ||||
Origin | Score | Origin | Score | Origin | Score | Origin | Score | |
SNF | 0.9790 | 0.9790 | 0.6255 | 0.5708 | 0.4121 | 0.4125 | 0.3440 | 0.3326 |
ANF | 0.9790 | 0.9790 | 0.6571 | 0.6009 | 0.4351 | 0.4378 | 0.3665 | 0.3524 |
SKF | 0.9790 | 0.9790 | 0.6143 | 0.6198 | 0.4106 | 0.5314 | 0.3281 | 0.3274 |
SRF | 0.9790 | 0.9790 | 0.6216 | 0.6198 | 0.4171 | 0.4236 | 0.3210 | 0.2356 |
PINS | - | 0.9790 | 0.8721 | − | − | − | − | − |
For the convenience of description, Origin is used to represent the matrix of original data feature, and Score is used to represent the matrix based on the sample specific score; and k is the number of clustering. Since PINS is a method that automatically selects the optimal number of clusters without generating a comprehensive information matrix, therefore, here PINS do not calculate SC in Table 3, and only the cluster number corresponding to PINS results is compared with other methods.
From Table 3, it can be seen that SNF and ANF are significantly better than SKF and SRF under unsupervised conditions, because SC takes similarity between samples as the main means to evaluate clustering results[20], and SNF and ANF integrate the similarity matrix of multi-omics samples nicely. However, SKF will eventually establish a sparse comprehensive information matrix under the influence of sparse cores. In the calculation of SC, the influence of a large number of 0 will dilute the numerical gap of inter-cluster distance and inter-cluster distance, so the SC measure is relatively poor in SKF. SRF has a model of associating the same sample data through regression model learning omics, which makes the obtained comprehensive information matrix have less information about sample similarity. Also, we can see that the score-based methods in the clustering number is 2 which is the real cluster number are better than using origin features in four methods on SC measure. It shows that method based on sample specificity scores can dig more information which reflect differences between samples, so as to better differentiate cancer subtypes.
From Tables 4 and 5, we can see that when the cluster number is 2, NMI and ARI are close to 1 in SNF, ANF, SKF and SRF methods, and sample specificity scores and original data can correctly classify samples with different cancer types. While PINS would misclassify some samples when using original data, but would be correct when using sample specificity scores. This further illustrates the advantage of sample specificity score in the identification of cancer subtypes. On the other hand, when the number of clusters is 3, 4 and 5 (when different cancer subtypes are further classified), the performance of the sample specificity score was better than the original data when the number of clusters is 4. When the number of clusters was 3 and 5, the performance of the sample specificity score is slightly worse or equal to the original data.
The sample survival probability curve can analyze the survival probability of multiple groups of samples in a certain period of time[21]. In medical research, cancer subtype survival analysis is often used as the clinical symptoms of cancer samples to determine the degree of malignancy and prognostic treatment options, which has guiding significance for the classification of cancer subtypes[22]. Cox log-rank p-value quantitatively evaluated the significance of clinical survival patterns in samples with different cancer subtypes[23]. In general, p-value<0.05 indicated that the subtype classification results are reasonable. Therefore, in order to reflect the performance of different methods in subtype identification, survival analysis is carried out on the clustering results with different clusters k (as shown in Table 6). When k=3, the p-values based on sample specific score are slightly larger than the original data in SNF, ANF and SKF; when k is 4 and 5, the p-values based on sample specific score in most of the methods are much smaller than the original data. In order to observe the survival curve more intuitively, the survival curves of different clusters are shown as in Figs. 2–6 (including p-values of Cox log-rank test).
Methods | k=2 | k=3 | k=4 | k=5 | ||||
Origin | Score | Origin | Score | Origin | Score | Origin | Score | |
SNF | 0.0005 | 0.0005 | 0.0012 | 0.0023 | 0.0067 | 0.0037 | 0.0130 | 0.0006 |
ANF | 0.0005 | 0.0005 | 0.0013 | 0.0020 | 0.0030 | 0.0063 | 0.0100 | 0.0006 |
SKF | 0.0005 | 0.0005 | 0.0016 | 0.0018 | 0.0028 | < 0.0001 | 0.0003 | 0.0001 |
SRF | 0.0005 | 0.0005 | 0.0031 | 0.0017 | 0.0180 | 0.0048 | 0.0170 | 0.0160 |
PINS | - | 0.0004 | 0.0022 | - | - | - | - | - |
In the SNF (Fig. 2), ANF (Fig. 3), SKF (Fig. 4) and SRF (Fig. 5) methods, when k=2, the survival analysis curves using original data and sample specificity scores are completely consistent. But in different clusters, further samples specificity scores on the survival curve performance better, have a clear area boundary. Specifically, when k=5, sample specificity scores in the four methods, has completely beyond the original data; when k=4 this phenomenon is more obvious in SKF method. In the PINS (Fig. 6) method, when sample specificity score is used, PINS automatically select two real result categories, while when the original data is used, cancer samples are automatically divided into three categories, and the differentiation degree of survival curve is worse than the sample specificity score. The above results indicate that the sample specificity score obtained by using the single-sample information gain strategy is significantly better than that obtained by using the original data for further identification of different cancer subtypes.
We know that four methods, SNF, ANF, SKF and SRF, are based on sample similarity, and generate a comprehensive information matrix between samples to describe the similarity relationship between samples. Finally, according to the comprehensive information matrix, cancer patients are divided into different clusters by clustering algorithm. It can be said that the performance of clustering algorithm will directly affect the accuracy of cancer subtype identification. The above four methods based on similarity fusion use the same spectral clustering algorithm in the end. The performance of spectral clustering algorithm mainly depends on the comprehensive information matrix. By comparing the comprehensive information matrix obtained by the four methods, we can directly compare the performance of samples specific scores obtained by SSIG model and original data.
In order to verify the advantage of the sample specificity score obtained by SSIG based on single sample in the identification of cancer subtypes, we use the comprehensive information matrix between samples and comprehensively arrange all samples according to the cancer subtype label obtained by k=2,3,4 and 5 respectively, to draw the sample similarity heat map. The deeper the color of the heat map of sample similarity, the higher the similarity between samples. The more distinct the difference areas in the heat map, and the more hierarchical the heat map. The more conducive the comprehensive information matrix is to cluster analysis and the easier it is to obtain accurate cancer subtypes. Because of different methods for comprehensive information matrix values range have bigger difference, the data is normalized for each method, shown in Fig. 7. It is shown that the boundary of similarity heat map clustering of the original data is relatively fuzzy, while the similarity heat map clustering edge of the sample specificity score is relatively clearer in the four methods. It can also be observed that when k=5 in the SNF (Fig. 7(a)), ANF Fig. 7(b)) and SKF (Fig. 7(c)) methods, the Cluster 2 (green cluster) would further divide the samples into two clusters, which is the reason for the significant reduction of Cox p-value in survival analysis. Especially in SKF, when k=4, small clusters will be further divided, so its Cox p-value is very significant. In the SRF (Fig. 7(d)) method, compared with the sample specificity score, there are a large number of yellow areas outside the similarity heat map clustering area of the original data, which will seriously affect the accuracy of identification of cancer subtypes. Therefore, when k=5, the Cox p-value of SRF shows the worst performance. From what has been discussed above, we believe that the sample specificity score can eliminate redundant information between samples to reflect more differences between samples and perform better in the identification of cancer subtypes.
In Fig. 7, the comment line above the similarity heat map (Cluster 2–5) is the cancer subtype label obtained by using different data in different methods. The comprehensive information matrix obtained by SKF is a sparse matrix, so there are very few dark regions outside the clustering region. SRF obtained a comprehensive information matrix after regression correction, so there are a lot of linear regions outside the cluster region.
Considering the current emergence of multi-omics data, the approaches of identifying cancer subtypes by fusing multi-omics data were proposed. To reflect the influence of individual sample specificity on cancer subtype identification, we propose a strategy based on SSIG to obtain the sample feature matrix, namely multi-omics sample specific scores, and compare the performance of the sample original feature matrix in subtype. First, three omics data, gene expression, DNA methylation and miRNA data of BRCA and KIRC are used. Then calculate sample specificity scores for each feature and each sample using SSIG model. Secondly, on the basis of the data, the multi-omics sample specificity score is applied to five classical methods of cancer subtypes (SNF, ANF, SRF, SKF, PINS), and some cancer subtype labels were obtained. By comparing the original data with the score data on three clustering measures (SC, NMI, ARI), survival analysis and comprehensive information matrix of the heat map, it is shown that subtype identification based on sample specific scores is more effective than that based on original data.
Heterogeneity is a characteristic of cancers. That is to say, within the scope of a cancer, different patients do not have a single tumor characteristic, but have their own relatively specific characteristics and clinical manifestations, which makes different patients have differences in drug sensitivity, radiotherapy sensitivity and other aspects. This also makes a difference in the treatment and prognosis of tumors. Through considering the specificity between samples, the omics data analysis and the identification of cancer subtypes for each sample are of great significance for discovering the specificity between samples. This method based on sample specificity can also be applied to identify and analyze disease markers of individual samples and conduct personalized treatment.
[1] |
Prasad V, Fojo T and Brada M, "Precision oncology: Origins, optimism, and potential", The Lancet Oncology, Vol. 17, No. 2, pp. 81-86, 2016. DOI: 10.1016/S1470-2045(15)00620-8
|
[2] |
Akbani R, Ng KS, Werner HM, et al., "A pan-cancer proteomic analysis of the cancer genome atlas (TCGA) project", Cancer research, Vol. 74, No. 19, pp. 4262-4262, 2014. http://cancerres.aacrjournals.org/content/74/19_Supplement/4262
|
[3] |
Zhao J, Xie XJ, Xu X, et al., "Multi-view learning overview: Recent progress and new challenges", Inform Fusion, Vol. 38, No. 2, pp. 43-54, 2017. http://www.sciencedirect.com/science/article/pii/S1566253516302032
|
[4] |
Rappoport N and Shamir R, "NEMO: Cancer subtyping by integration of partial multi-omic data", Bioinformatics, Vol. 35, No. 18, pp. 3348-3356, 2019. DOI: 10.1093/bioinformatics/btz058
|
[5] |
Suzan A, Sorin D and Nguyen. T, "Integrated cancer subtyping using heterogeneous genome-scale molecular datasets", Pac Symp Biocomput, Vol. 38, No. 25, pp. 551-562, 2020. http://www.researchgate.net/publication/338301137_Integrated_Cancer_Subtyping_using_Heterogeneous_Genome-Scale_Molecular_Datasets
|
[6] |
Rappoport N and Shamir R, "Multi-omic and multi-view clustering algorithms: Review and cancer benchmark", Nucleic Acids Research, Vol. 47, No. 2, pp: 1044-1044, 2019. DOI: 10.1093/nar/gky1226
|
[7] |
Wang B, Mezlini AM, Demir F, et al., "Similarity network fusion for aggregating data types on a genomic scale", Nature methods, Vol. 11, No. 3, pp: 333-337, 2014. DOI: 10.1038/nmeth.2810
|
[8] |
Ma T and Zhang A, "Affinity network fusion and semi-supervised learning for cancer patient clustering", Methods, Vol. 145, No. 8, pp. 16-24, 2018. http://europepmc.org/abstract/MED/29807109
|
[9] |
Guo Y, Zheng J, Shang X, et al., "A similarity regression fusion model for integrating multi-omics data to identify cancer subtypes", Genes, Vol. 9, No. 7, pp. 314-322, 2018. DOI: 10.3390/genes9070314
|
[10] |
Jiang L, Xiao Y, Ding Y, et al., "Discovering cancer subtypes via an accurate fusion strategy on multiple profile data", Frontiers in Genetics, Vol. 20, No. 5, pp. 10-20, 2019. http://www.ncbi.nlm.nih.gov/pubmed/30804977
|
[11] |
Nguyen T, Tagett R, Diaz D, et al., "A novel approach for data integration and disease subtyping", Genome Research, Vol. 27, No. 12, pp. 2025-2039, 2017. DOI: 10.1101/gr.215129.116
|
[12] |
Liu XP, Wang YT, Ji HB, et al., "Personalized characterization of diseases using sample-Specific networks", Nucleic Acids Research, Vol. 44, No. 22, pp. 164-164, 2016. DOI: 10.1093/nar/gkw772
|
[13] |
Rappoport N and Shamir R, "Multi-omic and multi-view clustering algorithms: Review and cancer benchmark", Nucleic Acids Research, Vol. 47, No. 2, pp. 1044-1044, 2019. DOI: 10.1093/nar/gky1226
|
[14] |
Duan R, Gao L, Xu H, et al., "CEPICS: A comparison and evaluation platform for integration methods in cancer subtyping", Frontiers in Genetics, Vol. 19, No. 10, pp. 966-978, 2019. http://www.ncbi.nlm.nih.gov/pubmed/31649733
|
[15] |
Cancer Genome Atlas Research N, Weinstein JN, Collisson EA, et al., "The cancer genome atlas pan-cancer analysis project", Nature Genetics, Vol. 45, No. 10, pp. 1113-1120, 2013. DOI: 10.1038/ng.2764
|
[16] |
Hidalgo SJT and Ma SG, "Clustering multilayer omics data using muncut", BMC Genomics, Vol. 19, No. 1, pp. 198-198, 2018. DOI: 10.1186/s12864-018-4580-6
|
[17] |
Peter J. Rousseeuw, "Silhouettes: A graphical aid to the interpretation and validation of cluster analysis", Journal of Computational and Applied Mathematics, Vol. 20, No. 1, pp. 53-65, 1987. http://www.sciencedirect.com/science/article/pii/0377042787901257
|
[18] |
Estevez PA, Tesmer M, Perez CA, et al., "Normalized mutual information feature selection", IEEE Transactions on Neural Networks, Vol. 20, No. 2, pp. 189-201, 2009. DOI: 10.1109/TNN.2008.2005601
|
[19] |
Steinley D, "Properties of the Hubert-Arabie adjusted Rand index", Psychological Methods, Vol. 9, No. 3, pp. 386-396, 2004. DOI: 10.1037/1082-989X.9.3.386
|
[20] |
Richardson M, Garner P and Donegan S, "Cluster randomised trials in cochrane reviews: Evaluation of methodological and reporting practice", PloS one, Vol. 11, No. 3, pp. 53-65, 2016. http://europepmc.org/articles/PMC4794236/
|
[21] |
Zhang SL, Wang X, Li ZM, et al., "Score for the overall survival probability of patients with first-diagnosed distantly metastatic cervical cancer: A novel nomogram-based risk assessment system", Frontiers in Oncology, Vol. 5, No. 9, pp. 1106-1106, 2019. http://www.ncbi.nlm.nih.gov/pubmed/31750238
|
[22] |
Henshall SM, Afar DE, Hiller J, et al., "Survival analysis of genome-wide gene expression profiles of prostate cancers identifies new prognostic targets of disease relapse", Cancer Research, Vol. 63, No. 14, pp. 4196-4203, 2003. http://carcin.oxfordjournals.org/cgi/ijlink?linkType=ABST&journalCode=canres&resid=63/14/4196
|
[23] |
Shi Q, Zhang C, Peng M, et al., "Pattern fusion analysis by adaptive alignment of multiple heterogeneous omics data", Bioinformatics, Vol. 33, No. 17, pp. 2706-2714, 2017. DOI: 10.1093/bioinformatics/btx176
|
1. | Naik, J.B., Kalli, S.N.R., Boda, R. Comparative analysis of image classification with retrieval system. International Journal of Ad Hoc and Ubiquitous Computing, 2023, 42(4): 226-242. DOI:10.1504/IJAHUC.2023.130463 |
2. | Siva Krishna, G., Prakash, N. A new training approach based on ECOC-SVM for SAR image retrieval. International Journal of Intelligent Enterprise, 2021, 8(4): 492-517. DOI:10.1504/IJIE.2021.117992 |
3. | Jin, G., Zhang, Y., Lu, K. Deep hashing based on VAE-GaN for efficient similarity retrieval. Chinese Journal of Electronics, 2019, 28(6): 1191-1197. DOI:10.1049/cje.2019.08.001 |
4. | Naga Raju, T., Suneetha, C. Feature extraction and content based image retrieval for high resolution remote sensing images. International Journal of Recent Technology and Engineering, 2019, 8(3): 8877-8880. DOI:10.35940/ijrte.C6677.098319 |
5. | Raghuwanshi, G., Tyagi, V. Feed-forward content based image retrieval using adaptive tetrolet transforms. Multimedia Tools and Applications, 2018, 77(18): 23389-23410. DOI:10.1007/s11042-018-5628-y |
6. | Mathan Kumar, B., PushpaLakshmi, R. Multiple kernel scale invariant feature transform and cross indexing for image search and retrieval. Imaging Science Journal, 2018, 66(2): 84-97. DOI:10.1080/13682199.2017.1378285 |
7. | Ali, A., Sharma, S. Content based image retrieval using feature extraction with machine learning. 2017. DOI:10.1109/ICCONS.2017.8250625 |
Diseases | mRNA | DNA methylation | miRNA | |||
Genes | Samples | CpG sites | Samples | miRNAs | Samples | |
BRCA | 20531 | 1104(114) | 27579 | 318(27) | 2239 | 756(76) |
KIRC | 20531 | 534(72) | 27579 | 219(199) | 2049 | 241(70) |
Diseases | mRNA | DNA methylation | miRNA | |||
Genes | Samples | CpG sites | Samples | miRNAs | Samples | |
BRCA | 17487 | 130(114) | 20417 | 130(27) | 247 | 130(76) |
KIRC | 17487 | 63(72) | 20417 | 63(199) | 247 | 63(70) |
Methods | k=2 | k=3 | k=4 | k=5 | ||||
Origin | Score | Origin | Score | Origin | Score | Origin | Score | |
SNF | 0.9817 | 0.9974 | 0.8120 | 0.6320 | 0.7278 | 0.6514 | 0.6563 | 0.4793 |
ANF | 0.8767 | 0.9820 | 0.8491 | 0.4722 | 0.5853 | 0.5456 | 0.5167 | 0.3260 |
SKF | 0.3698 | 0.4270 | 0.5502 | 0.4385 | 0.6034 | 0.4978 | 0.5826 | 0.5325 |
SRF | 0.0154 | 0.2258 | 0.0059 | 0.1265 | 0.0043 | 0.1028 | 0.0028 | 0.0476 |
Methods | k=2 | k=3 | k=4 | k=5 | ||||
Origin | Score | Origin | Score | Origin | Score | Origin | Score | |
SNF | 0.9547 | 0.9547 | 0.7480 | 0.7317 | 0.6494 | 0.6495 | 0.6074 | 0.6067 |
ANF | 0.9547 | 0.9547 | 0.7874 | 0.7697 | 0.6817 | 0.6826 | 0.6381 | 0.6349 |
SKF | 0.9547 | 0.9547 | 0.7445 | 0.7462 | 0.6489 | 0.6808 | 0.6054 | 0.6052 |
SRF | 0.9547 | 0.9547 | 0.7194 | 0.7462 | 0.5883 | 0.6531 | 0.5302 | 0.4528 |
PINS | − | 0.9547 | 0.8594 | − | − | − | − | − |
Methods | k=2 | k=3 | k=4 | k=5 | ||||
Origin | Score | Origin | Score | Origin | Score | Origin | Score | |
SNF | 0.9790 | 0.9790 | 0.6255 | 0.5708 | 0.4121 | 0.4125 | 0.3440 | 0.3326 |
ANF | 0.9790 | 0.9790 | 0.6571 | 0.6009 | 0.4351 | 0.4378 | 0.3665 | 0.3524 |
SKF | 0.9790 | 0.9790 | 0.6143 | 0.6198 | 0.4106 | 0.5314 | 0.3281 | 0.3274 |
SRF | 0.9790 | 0.9790 | 0.6216 | 0.6198 | 0.4171 | 0.4236 | 0.3210 | 0.2356 |
PINS | - | 0.9790 | 0.8721 | − | − | − | − | − |
Methods | k=2 | k=3 | k=4 | k=5 | ||||
Origin | Score | Origin | Score | Origin | Score | Origin | Score | |
SNF | 0.0005 | 0.0005 | 0.0012 | 0.0023 | 0.0067 | 0.0037 | 0.0130 | 0.0006 |
ANF | 0.0005 | 0.0005 | 0.0013 | 0.0020 | 0.0030 | 0.0063 | 0.0100 | 0.0006 |
SKF | 0.0005 | 0.0005 | 0.0016 | 0.0018 | 0.0028 | < 0.0001 | 0.0003 | 0.0001 |
SRF | 0.0005 | 0.0005 | 0.0031 | 0.0017 | 0.0180 | 0.0048 | 0.0170 | 0.0160 |
PINS | - | 0.0004 | 0.0022 | - | - | - | - | - |