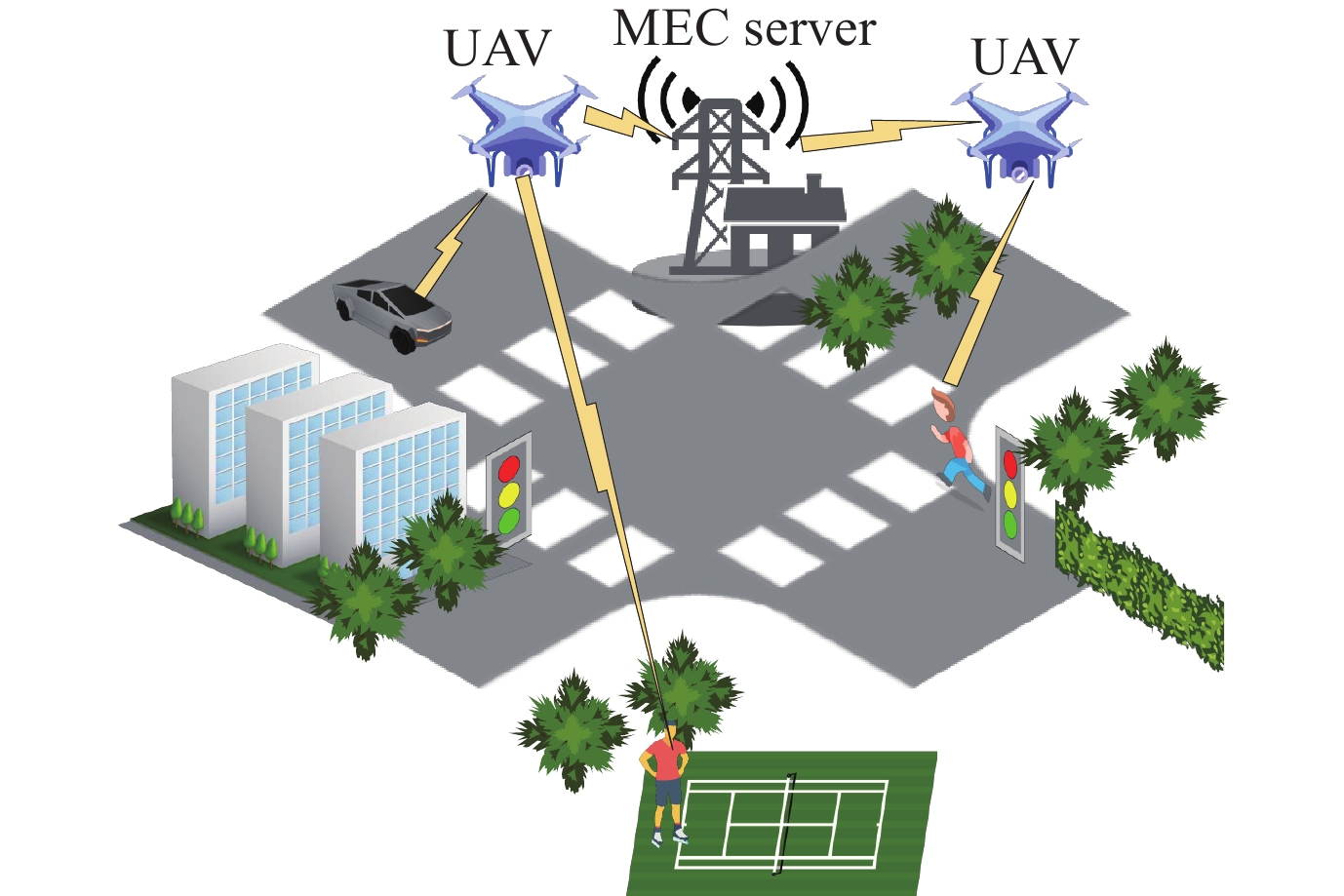
Citation: | Shancheng Zhao, Wantong Zhao, Xin Fan, et al., “When RIS and digital twin meet uav-assisted mobile edge computing: a survey,” Chinese Journal of Electronics, vol. x, no. x, pp. 1–15, xxxx. DOI: 10.23919/cje.2024.00.229 |
In the ultra 5G/6G era, unmanned aerial vehicle (UAV)-assisted mobile edge computing (AEC) emerges as a vital component in the internet of things (IoT). By leveraging the flexible deployment capabilities of UAVs, AEC offers users low-latency and high-bandwidth edge computing services. However, the progression of AEC networks still confronts serious challenges, including channel blockages, complex channel fading, and real-time decision requirements. To effectively address these challenges, the emerging reconfigurable intelligence surface (RIS) and digital twin (DT) technologies demonstrate immense optimization potential. Specifically, RIS enhances the efficiency and reliability of computational offloading by optimizing the intricate UAV communication environment. Simultaneously, DT technology digitizes wireless networks, facilitating low-cost, highly adaptive, and rapid optimization and design of AEC networks. These two technologies open up a new path for the advancement of AEC networks. To our knowledge, there is a lack of comprehensive reviews addressing this emerging cross-cutting area. Therefore, this paper aims to fill this gap by providing an in-depth analysis of the latest research progress of RIS and DT technologies-assisted AEC networks. Ultimately, this paper furnishes a clear and comprehensive perspective, exploring the immense potential of RIS and DT technologies in driving the development of AEC networks.
With the advent of the ultra 5G/6G era and the rapid development of the internet of things (IoT), the count of IoT user devices is projected to surge to 50 billion by 2030 [1]. This result poses challenges with computational demands, latency, and data transmission. To tackle these challenges, mobile edge computing (MEC) technology emerges as a solution. MEC provides a cloud-like service to address computational constraints by deploying computational resources at the network’s edge [2], [3]. This deployment enables devices to access computing resources more quickly and reliably, enhancing user experience and mitigating network congestion during data transmissions [4], [5]. However, traditional MEC’s fixed deployment limits coverage, causing uneven resource allocation and communication disruptions, especially in remote areas.
To further enhance the performance of MEC, UAV technology is a key integration technology. Figure 1 illustrates the network model of UAV-assisted edge computing (AEC). In MEC networks, UAVs can serve as computational nodes or relay stations, collaborating with edge servers to provide the entire network with more flexible and versatile task processing capabilities [6]. Furthermore, unlike traditional ground-based servers, UAVs operating in the sky are accessible from the constraints of complex terrain on the ground, significantly expanding network coverage and increasing the likelihood of line-of-sight communication [7]. Simultaneously, UAVs can flexibly adjust their positions based on the real-time offloading needs of devices, further optimizing the offloading experience [8]. Currently, AEC networks demonstrate their unique value in various application fields.
Specifically, in the internet of vehicles (IoV), traditional network architectures often suffer from significant service delays due to limited computing resources and network congestion [17]. In response to this challenge, in [18], the authors propose a UAV-assisted vehicular ad hoc networks (VANETs) architecture supporting MEC. In this architecture, UAVs can flexibly provide computing services to multiple vehicles, effectively reducing the computational load on ground vehicles. The authors in [19] establish a MEC network model encompassing UAVs, vehicles, and roadside units. They employ long short-term memory (LSTM) networks and attention mechanisms to address the dynamics of task arrivals, significantly reducing system latency in the IoV. Additionally, the authors in [20] introduce UAVs to address the overload issue of roadside computing units in vehicle-congested areas and propose an online offloading algorithm based on Markov approximation optimization.
In complex terrains like mountainous areas and disaster zones, AEC networks similarly hold an indispensable position. By integrating MEC technology, UAVs offer efficient remote sensing monitoring and autonomous decision-making capabilities for hard-to-reach areas, which are vital for swift response and disaster management [21]. Meanwhile, by carefully planning the trajectories of UAVs and effectively utilizing MEC technology, rescue teams can quickly establish a network and allocate necessary computing resources during disasters, providing solid and flexible support for rescue operations [22]. Additionally, AEC can be seamlessly integrated with vehicle fog computing (VFC) to construct a three-tier disaster relief computing architecture, markedly enhancing the overall system’s performance [23]. Though the AEC network has many advantages, its practical implementation poses several challenges. Complex and ever-changing environmental factors can introduce obstructions and signal degradation in the air-to-ground channel, affecting the reliability of data transmission. Furthermore, the long distance of line-of-sight links and the UAVs' limited computational resources adversely affect the delay-sensitive tasks.
Reconfigurable intelligence surface (RIS) represents an advanced technology designed to optimize wireless network communication environments. In AEC networks, RIS can reshape complex and dynamic wireless channel environments by precisely controlling the amplitude and phase of incident signals [24]. This capability not only effectively mitigates signal attenuation and interference but also significantly enhances uplink transmission rates, thereby enabling efficient computation task offloading [25]. Furthermore, authors in [26] explore the potential of deploying RIS on a UAV. They propose an iterative algorithm to optimize the base station’s beamforming and RIS phase shift matrix, resulting in a 50% increase in system energy efficiency. However, it is noteworthy that RIS-assisted AEC networks pose higher demands for real-time channel optimization, resource allocation, and computation task scheduling due to their high degrees of dynamism and complexity.
Digital Twin (DT) technology plays a pivotal role in AEC networks, owing to its unique capability of bridging the physical and virtual worlds. DT technology enables a comprehensive digital mapping of AEC networks, thereby facilitating cost-effective, adaptable, and rapid optimization and design of the entire network. Authors in [27] focus on the intelligent deployment and optimal resource allocation of UAVs, proposing a digital twin-assisted and density-aware deployment strategy. This approach innovatively tackles the complexity of task divisibility and data interdependencies, offering a fresh perspective on resource scheduling in complex environments. Furthermore, authors in [28] propose a DT-driven and RIS-assisted edge computing system, leveraging a DT-assisted deep reinforcement learning algorithm to reduce transmission delay and optimize energy consumption.
To summarize, the broad prospects of RIS and DT technologies are attracting deep attention and extensive discussions in academia and industry. Table 1 summarizes some reviews about RIS, DT, and AEC. However, these reviews predominantly concentrate on the separate applications or partial integrations of UAVs, RIS, DT, and MEC technologies. Specifically, reviews [9] and [10] establish the groundwork for deploying UAVs in MEC, yet neither considers the research status of RIS and DT technologies within this context. Similarly, the review [11] focuses on the role of UAVs in 6G networks without considering RIS and DT technologies. In terms of RIS research, reviews [12] and [13] underscore the potential of RIS to enhance air-ground network performance, but they lack integration and analysis with MEC and DT. Meanwhile, the review [14] discusses the challenges and opportunities of aerial RIS in MEC networks, but it does not provide a comprehensive summary of current RIS research in AEC and neglects the role of DT technology. Furthermore, reviews [15] and [16] delve into the key characteristics of DT networks and their application scenarios in 6G, but neither considers UAVs or RIS. In summary, there is currently a gap in the comprehensive summary of RIS and DT technologies in UAV-assisted edge computing systems. Given this, this paper aims to offer valuable reference and guidance for in-depth research in integrating RIS and DT technologies with AEC, through a comprehensive summary and analysis of existing research findings. The key contributions of this paper are outlined as follows:
Reference | Contribution | RIS | DT | UAV | MEC |
[9] | Review on the architecture, challenges, performance metrics, and applied state-of-the-art technologies for aerial edge computing | ||||
[10] | Survey on the benefits, challenges, and research advances in UAV-enabled edge computing | ||||
[11] | Comprehensive review of the use of UAVs in 6G networks, emphasizing their utility in mission offloading and data latency challenges | ||||
[12] | Survey on the advantages and potential applications of RIS in UAV support systems | ||||
[13] | Survey on the opportunities and related challenges of integrating UAV and RIS technologies for enhancing air-ground network performance | ||||
[14] | Review on how aerial RIS improves the performance of mobile edge computing networks and discusses challenges and opportunities | ||||
[15] | Review on the main features, key technologies, challenges, application scenarios and new research dynamics of digital twin networks | ||||
[16] | Survey on the application, design, typical scenarios, and challenges faced by digital twin edge networks in 6G | ||||
This paper | Survey on the advancements, challenges, potential, and new opportunities of RIS and digital twin technologies in UAV-assisted edge computing systems |
Pioneering review: This paper innovatively conducts a comprehensive analysis of the integration of RIS and DT within AEC, filling a gap in systematic reviews. It aims to guide the future development of the AEC field by summarizing research progress and highlighting potential issues.
Comprehensive analysis and multi-dimensional summary: Unlike previous related reviews, this paper provides a more detailed and profound summary and analysis. We have constructed a multi-layered framework that encompasses network architecture, optimization objectives, unresolved challenges, and applications. This framework elucidates the latest advancements and fosters a deeper understanding of the field.
Exploration and future perspectives: Based on a comprehensive review of current research, this paper delves into the innovative potential of integrating RIS, DT, and AEC, opening up new research pathways and identifying key challenges. Furthermore, we point the way forward for future research efforts by posing a series of questions that warrant further investigation.
The remainder of this paper is structured as shown in Figure 2. Section 2 introduces the critical technologies of RIS and DT. Section 3 explores the research progress of RIS-assisted AEC, including introducing network architectures, analyzing research content across various performance metrics, and revealing unresolved issues. Furthermore, we discuss the potential application scenarios. Section 4 explores the research progress of DT-supported AEC. Section 5 broadens the research horizon by delving into the integration of RIS, DT, and AEC, analyzing the possibilities and the challenges it faces. Section 6 looks forward to future research directions. Section 7 summarizes the entire paper.
In this section, we summarize the pivotal technologies of RIS and DT.
RIS emerges as an innovative technology and is regarded as a revolutionary enabler of an intelligent radio environment. It intelligently reconfigures wireless paths and mitigates adverse effects in communication transmission.
Specifically, RIS typically consists of a two-dimensional array of passive reflective elements based on technologies such as liquid crystals, varactor-tuned resonators, and electromechanical switches. The existing RIS designs are diverse, yet most focus on a three-layer architecture [29]: (1) the metasurface layer, composed of passive conductive elements; (2) the control layer, responsible for altering the amplitude or phase of these elements; and (3) the gateway layer, facilitating communication between the control layer and other devices. Intelligently, each element modulates the electromagnetic properties of subwavelength scatterers. This capability enables RIS to perform functions like beam steering, filtering, polarization, and shaping.
Based on the in-depth analysis of existing articles, we can classify the existing RIS into the following three categories.
Passive RIS:This type of RIS typically only utilizes low-power adjustable components such as PIN diodes or MEMS switches. These components require only minimal energy to drive, thus enabling intelligent phase modulation of signals without the need for an external power supply. It modulates signals solely through intelligent phase control of its reflectors. However, in environments with high fading coefficients, the path loss of multiple segments leads to significant signal power depletion.
Active RIS: Equipped with built-in phase-shifting circuits and reflective amplifiers, active RIS exerts precise control over the phase of reflected signals while significantly boosting their intensity. This effectively mitigates the fading issues inherent in passive RIS systems. However, it is important to note that Active RIS comes with a higher power consumption requirement.
STAR-RIS: The majority of traditional RIS designs are confined to single-sided reflection, catering to users within a hemispherical area. Conversely, STAR-RIS is a new type of intelligent surface that can transmit and reflect signals concurrently, thereby enabling comprehensive 360° space coverage. It boasts the flexibility to serve both transmitting and receiving users simultaneously through three protocols: energy splitting, mode switching, and time switching [30].
Based on their lifetime length and the flow of data within digital twins, we can categorize them into two categories:
Digital Twin Prototype (DTP): It intervenes in the system during its design phase, with the core integrating critical data and information, enabling a seamless transition from virtual to physical. Typically, the DTP operates as a static mirror or unidirectional data flow model, supporting multiple rounds of testing to safeguard design integrity and system stability.
Digital Twin Instance (DTI): It is enabled during the operational phase of the system, enabling a two-way flow of data and tight synchronization with physical entities. It both monitors physical system behavior and guides optimization decisions, ensuring that the system operates as expected and efficiently troubleshoots potential failures.
According to different application scenarios and performance requirements, we can divide the deployment strategy of DT into two categories.
Static Deployment: This approach establishes a stable operating DT system at a fixed location. The crux lies in selecting the most suitable deployment site according to application needs and ensuring that the DT operates stably and efficiently at that location. Depending on specific application demands and scenario characteristics, the DT can usually be deployed on cloud servers, edge servers, or physical entities.
Dynamic Deployment: This strategy emphasizes the capability of the DT to migrate as the physical object moves flexibly. As a virtual representation of the physical entity, the DT dynamically shifts from one edge server to another in response to changes in the physical entity’s location, ensuring precise and real-time data feedback.
This section overviews the network architecture, current research findings, unresolved challenges, and potential application scenarios within the field of RIS-assisted AEC.
As depicted in Figure 3, we categorize RIS devices into two types based on their deployment: the terrestrial RIS (TRIS) and the UAV equipped with RIS (ARIS). TRIS devices are pre-positioned at fixed locations, either on the ground or mounted on buildings. They facilitate communication for ground terminals with obstructed links, enabling them to transmit tasks to UAVs and distant base stations. This arrangement extends network coverage and significantly enhances the propagation environment.
Furthermore, the ARIS constitutes a dynamic RIS network framework. In this configuration, RIS can be flexibly deployed and adjusted in the air, enabling it to adapt to changing environmental conditions and optimize communication and transmission performance. Additionally, the UAV can act as an edge server to offer terminals more offloading opportunities, further enhancing the system’s quality of service. Notably, the dynamic RIS has a better full-angle beamforming capability than the static RIS.
In AEC systems, where the energy and computational resources of UAVs and various devices are limited, energy efficiency is a critical research priority. Utilizing RIS technology and innovative optimization strategies provides new ways to reduce energy consumption in UAVs and terminal devices. Specifically, the study in [31] utilizes the phase adjustment capabilities of RIS to enhance UAV-ground communication, enabling efficient task offloading to edge servers. Meanwhile, the authors use the double deep Q-network (DDQN) algorithm to solve complex non-convex optimization problems, thereby identifying optimal resource allocation and task offloading strategies that minimize UAV energy consumption. Similarly, The authors in [32] further propose an innovative dual-RIS architecture for vehicular networks, strategically placing RISs near vehicles and ground road side units. By integrating UAVs as server nodes and relays, this architecture optimizes signal paths, reducing signal attenuation and delays. Furthermore, the authors utilize the Lagrangian duality and subgradient methods for optimizing power allocation, time slot scheduling, and task assignment to minimize weighted total energy consumption. In [33], RISs are deployed on surrounding building walls to support task offloading, combined with partial offloading and non-orthogonal multiple access (NOMA) to serve multiple IoT devices efficiently via UAV-mounted MEC servers. This approach improves spectrum utilization, reduces IoT device transmission power, and accelerates task completion. The authors also design a two-layer iterative algorithm based on Dinkelbach and block coordinate descent (BCD) to maximize energy efficiency.
Furthermore, the study [34] explores UAV-mounted RISs to assist communication between ground users and edge servers, treating UAVs solely as signal reflection platforms. By jointly optimizing UAV trajectories, RIS phase shifts, and edge resource allocation, this study achieves efficient energy utilization and enhanced system energy efficiency. Similarly, the study in [35] investigates the use of UAVs equipped with RIS to enhance communication for inland river vessels. The reflective properties of RIS facilitate the offloading of computational tasks from unmanned surface vehicles (USVs) to MEC servers, thereby reducing energy consumption. Meanwhile, the authors employ an improved block coordinate descent algorithm to jointly optimize offloading decisions, transmission power, UAV trajectories, and RIS phase adjustments. Furthermore, the study in [36] proposes a novel MEC system where UAVs equipped with RISs selectively relay or process IoT device tasks, enhancing system adaptability. Additionally, for scenarios where RIS elements have the same or different phase shifts, the study proposes graph theory-based solutions and random search algorithms to minimize system energy consumption. In [37], the authors introduce a dual-UAV collaborative MEC system integrating aerial RIS and aerial MEC servers. The RIS dynamically adjusts phase shifts to optimize communication channels, while the MEC server allocates computational resources flexibly. The system leverages the DDQN algorithm for real-time optimization of UAV trajectories, RIS configurations, and resource scheduling, significantly improving energy efficiency in dynamic environments. Similarly, [38] explores aerial RIS as a communication relay and proposes a deep deterministic policy gradient (DDPG) algorithm to balance the costs of IoT device operations. Additionally, in [39], the authors innovatively propose a multi-RIS and multi-UAV collaborative MEC system architecture that improves communication quality and expands network coverage. The study introduces a phased optimization strategy to sequentially optimize UAV trajectories, user association, and RIS phase shifts, thereby minimizing operational costs.
The aforementioned research findings not only enrich the theoretical framework for optimizing the energy efficiency of RIS-assisted AEC systems but also provide technical guidance for practical deployments. However, we are also aware of some issues in the current research:
RIS phase shift adjustment energy consumption: Current studies ignore RIS energy consumption during phase-shift adjustment, especially unjustified for UAV-mounted RIS. Due to limited UAV energy resources, the RIS phase-shift adjustment strategy should be considered in overall energy consumption. Considering and optimizing RIS energy consumption within an energy efficiency framework is crucial for system stability and efficiency.
Real-time optimization under dynamic channel conditions: Most studies use idealized static models and neglect real-world channel dynamics and complexity. Real-time channel state sensing and dynamic RIS phase shift adjustment strategies are crucial for energy efficiency. This requires integrating advanced algorithm design with emerging technologies for accurate predictions and responses to channel states.
Computing capacity is a vital optimization target in AEC networks, representing the system’s ability to process and analyze data. In [40], the authors consider employing UAVs as eavesdroppers and utilizing RIS to enhance communication links between legitimate UAVs and users. Furthermore, the study jointly optimizes frame length, transmit power, local computing data allocation, UAV trajectories, and RIS phase shifts, thereby enhancing secure computing capabilities. Additionally, in [41], the authors leverage the phase control characteristics of RIS to enhance the signal strength of a power beacon (PB), thereby effectively improving the energy harvesting efficiency. Meanwhile, the study employs a multi-UAV collaborative approach to provide broader coverage and more flexible services and proposes an alternate search method (ASM) to maximize the total computational bit rate of IoT devices.
Furthermore, the authors in [42] integrate RIS with NOMA technology. RIS enhances signal phases, while NOMA improves resource allocation through power domain multiplexing. The authors also propose a joint optimization algorithm that collaboratively designs RIS phase shifts, NOMA power allocation, user decoding order, and UAV deployment to maximize UAV computational capacity. In challenging scenarios and harsh environments, RIS also demonstrates its effectiveness. In [43], the study addresses the issue of mountainous terrain by utilizing ground-RIS to enable communications to bypass obstacles and enhance communication quality. Additionally, the authors design a model-predictive control (MPC)-based scheduling algorithm for optimizing task execution.
Based on the research outlined above, it is evident that RIS plays a pivotal role in various aspects of optimizing the computational capabilities of AEC networks. Concurrently, we identify issues in current research:
The Security Potential of RIS: The communication of UAVs is vulnerable to security threats such as eavesdropping [44], [45]. However, the investigation into edge network security solutions leveraging RIS remains insufficient. Indeed, RIS holds immense potential in bolstering the security of AEC networks. Specifically, by intelligently adjusting the phase shifts of passive elements on the RIS, the reflected signals can coherently combine with signals from other paths at the desired receiver to enhance the received signal power while canceling signals at non-intended receivers to suppress interference effectively [46]. Therefore, exploring the potential application of RIS further in AEC networks is necessary.
Lack of research on multi-hop RISs: The application of single-sided RIS in AEC is limited by signal coverage and adaptability to dynamic environments, which affects data transmission. To address this challenge, Multi-Hop RIS technology emerges as a solution. Adding multiple nodes not only achieves broader signal coverage and higher data throughput but also effectively reduces signal attenuation and enhances the robustness of edge networks. Additionally, this technology can dynamically select the optimal transmission path and optimize resource allocation and power control, thereby significantly improving network energy efficiency and users' experience. Therefore, Multi-Hop RIS is a crucial research direction for enhancing AEC performance.
RIS-assisted AEC networks are widely recognized for their ease of deployment and high-efficiency communication. This subsection explores its potential application scenarios, including smart cities and disaster areas.
The smart city integrates diversified fields, such as the Internet of Things, smart grids, and buildings, to form an integrated network system. In this context, MEC networks assisted by RIS have become essential for development. Specifically, the RIS and UAVs equipped with RIS effectively reduce signal blind zones in the city, improve signal transmission quality, and mitigate signal interference. UAVs loaded with RIS can facilitate measurements, aiding urban planning and digital transformation. In conclusion, RIS-assisted and UAV-supported MEC networks enhance smart city manageability, adaptability, and control [47].
In disaster areas, network infrastructure such as base stations may often damaged, disrupting wireless connectivity and degrading signal quality, challenging management and rescue operations. Considering the stringent requirements of low latency and high sensitivity for disaster area management and rescue, UAVs with the assistance of RIS show unique advantages. Specifically, UAVs loaded with RIS achieve efficient information exchange between the disaster area and the outside world through air-to-ground links and full-angle reflections [48]. Furthermore, ARIS can intelligently assist users in dynamically transferring computational tasks to other edge servers, ensuring rescue efficiency. The integrated application of UAVs and RIS provides robust support for disaster rescue, highlighting their vital role and potential.
In this section, we overview the current research advancements of DT-driven AEC, including the introduction of the network architecture, a summary of existing research, and a discussion of potential application scenarios.
Figure 4 illustrates the MEC network architecture assisted by DT and UAV. The architecture has two main core components: the physical and digital twin layers. The physical layer encompasses all physical entities within the system and their intricate wireless communication environments. Meanwhile, the digital twin Layer consists of digital representations of various entities, digital communication models, and other relevant components. These digital twins store the physical entities' fundamental information and synchronize the system’s operational status in real time. Concurrently, the inter-twin interfaces facilitate the sharing and exchange of global information, empowering the digital twin layer to simulate, predict, and optimize the offloading scheme [49]. Notably, UAVs can play multiple roles in this architecture, such as essential relay nodes in the digital twin update process and critical providers of edge computing services.
In the B5G/6G era, a surge of latency-sensitive task requirements emerge. Achieving low latency reduces the operational time costs associated with UAVs and presents novel avenues for addressing challenges such as limited battery capacity. In [50], the authors deploy DTs at base stations to monitor network dynamics in real-time and optimize offloading strategies, resource allocation, and UAV trajectories. Using an iterative algorithm combining Lagrangian duality and successive convex approximation (SCA), the study minimizes the maximum task completion time of IoT devices. Similarly, the authors in [51] propose a DT-driven framework that integrates UAV-mounted MEC servers to reduce data transmission distances and latency. The framework further optimizes system performance by creating Digital Twins of IoT devices and MEC servers to predict processing rates and adjust offloading strategies dynamically. Simultaneously, it also employs short-packet transmission and efficient coding to meet the stringent latency and reliability demands of mission-critical services. Expanding the application of digital twins, the study [52] constructs an aerial network using multiple UAVs to collect real-time network state and traffic pattern data. The study proposes the RADiT algorithm, which is based on multi-network deep reinforcement learning and schedules resources across three computation modes. In addition, the study [53] designs a framework for UAV-ground vehicle collaboration, which creates cloud-based DT replicas of vehicles and uses SAGINs' communication architecture to ensure stable connectivity in high-speed scenarios. By employing a multi-agent deep reinforcement learning algorithm, the system dynamically adjusts task offloading and resource allocation, reducing latency in high-speed and distributed network scenarios.
To enhance communication resource utilization, [54] proposes a digital twin edge network (DTEN) model, combining UAVs and D2D communication with a multi-task deep reinforcement learning (DRL) method to jointly optimize relay node selection, bandwidth allocation, and UAV trajectories, minimizing network delays in high-density environments. Meanwhile, [55] develops a two-stage incentive mechanism using dynamic DTs and distributed algorithms to optimize task allocation, improving resource efficiency for space-air-ground integrated vehicular networks. Synchronization mechanisms represent another critical component for latency reduction in DT-based systems. Addressing the limitations of ground-based MEC systems, the study [56] designs a UAV-assisted synchronization framework that utilizes UAV mobility and line-of-sight links to efficiently collect sensor data from moving vehicles. The study employs an advantage actor-critic (A2C)-based resource allocation algorithm to reduce synchronization latency and improve task completion rates while minimizing UAV energy consumption. Additionally, in edge computing-assisted metaverse systems, the study [57] minimizes synchronization update latency by modeling dynamic UAV-sensed data and using a DDQN algorithm combined with transfer learning. This approach enhances generalization and adaptability in dynamic network conditions. Considering the resource and energy constraints of UAVs, the study [58] combines UAV trajectory and task allocation optimization with federated learning and deep reinforcement learning, improving system adaptability and minimizing delays and energy consumption.
To sum up, it is clear that DT exhibits a remarkable role in delay optimization within AEC systems. It significantly impacts aspects such as resource management, task offloading, communication resource allocation, and service quality optimization. However, we are also aware of some problems and challenges of the existing research:
Lack of DT update latency consideration: To ensure the synchronization between digital twins and the physical worlds, it must undergo frequent data update processes. Nevertheless, as the frequency of updates escalates, the resulting latency introduced by the DT directly impacts the efficiency and response time of system task offloading computations. To effectively address this challenge, we can explore an adaptive strategy that can intelligently adjust the update frequency of DT based on real-time changes in the system environment.
Neglect of the collaboration between edge and cloud Servers: In the current DT-driven AEC systems, research predominantly focuses on computation at the edge nodes. To fully utilize abundant computational resources on the cloud, we can establish multi-tier digital twins, enabling real-time optimization of multi-level task scheduling and resource allocation. This approach can significantly reduce task completion time.
The optimization of energy consumption remains crucial for improving UAV endurance and enhancing the quality of experience for users. By integrating real-time monitoring, virtual simulation, and dynamic optimization capabilities, DT can serve as an effective tool for increasing energy efficiency. In [59], the authors propose an adaptive task offloading framework, leveraging DT technology to monitor the network status and mobile trajectories of MTUs in real time. By combining a double deep Q-network (DDQN) algorithm with iterative optimization, it intelligently selects offloading nodes and methods while dynamically adjusting transmission power and resource allocation. This approach effectively reduces the overall energy consumption of the MEC system while meeting task delay requirements, providing a practical integration of DT with task offloading optimization. In addition, authors in [60] employ an incremental distributed update (IDU) mechanism to decentralize data analysis and modeling tasks to IIoT devices. By updating only changed sub-regions, the mechanism significantly reduces resource consumption at DT servers. Furthermore, the authors propose a self-adaptive trajectory decision (STD) scheme, allowing UAVs to adjust their paths based on real-time demands, thereby achieving higher energy efficiency.
To address the challenges of large-scale deep learning models in multi-UAV systems, the authors in [61] propose a lightweight model-sharing scheme based on DT. Edge DT constructs lightweight models and performs virtual simulations to determine the optimal model structure and network topology, thereby reducing computational and transmission resource consumption while improving recognition accuracy. Furthermore, to address the uncertainty in computational demands from mobile users, authors in [62] propose leveraging the real-time prediction capabilities of DT to optimize offloading decisions under uncertainty. This approach optimizes the offloading strategy by jointly considering factors such as mapping deviations between DT and actual network states, uncertain delays, and the number of idle UAVs.
DT exhibits remarkable efficacy in optimizing energy efficiency, particularly regarding DT deployment, communication optimization, and multi-UAV flight plans. Meanwhile, we also keenly identify some problems and challenges of the existing research:
Security Optimization: The communication security of AEC networks is susceptible to various threats, primarily due to signal interference and attack risks [63]. Currently, there is little research on using DT to assist the security of edge computing networks. In reality, DTs hold immense potential in strengthening the security of edge computing. We can utilize the real-time observation capability of DT to detect and respond to potential security issues in time to avoid ineffective task offloading. Meanwhile, we can use DT to simulate different security policies, evaluate their effectiveness and impact, and optimize the system’s security protection measures.
DT errors problem: Delayed state synchronization and transmission errors prevent digital twins from fully capturing the physical system’s real-time state [62]. This implies that there may be deviations in the simulations and predictions of digital twins, which could lead to inaccuracies in UAV trajectory planning and task allocation within the system, ultimately reducing the system’s overall energy efficiency. To address this, we propose designing and implementing an error monitoring and correction mechanism for digital twin models to minimize the impacts of errors on offloading strategy.
DT-driven AEC systems hold significant potential in various application scenarios, notably transportation and agriculture.
Within the transportation sector, to address transmission issues in regions with underdeveloped communication infrastructure, authors in [71] propose a system using UAVs and geosynchronous satellites. This system leverages DT technology to enhance solution quality while leveraging edge computing to reduce project costs and elevate technical performance. In this process, UAVs capture wireless sensor data and feed this real-time information into DT models. Additionally, the mini-edge computing platform proposed in [72] combines DT and MEC technologies, facilitating real-time urban traffic data acquisition and optimization. In summary, the combination of DT, UAV, and MEC can provide reliable and accurate service for comprehensive traffic analysis.
In agricultural applications, the Astoria platform introduced in [73] harnesses the synergy of UAV, DT, MEC, and artificial intelligence to forge a sustainable agriculture DT platform tailored for vineyards. This platform leverages 5G technology and edge computing to process high-resolution images captured by UAVs, crafting highly accurate DT models. Farmers can efficiently manage vineyards, optimize resource consumption, mitigate the risk of missed harvests, and enhance profitability with this platform.
Based on the integration status of RIS and DT within UAV-aided edge networks in prior sections, this subsection explores the possibility of integrating RIS and DT technology into AEC, proposes the network architecture, and analyzes the possible challenges it may encounter.
To comprehensively uncover the vast application prospects of RIS and DT, we systematically review the latest research achievements and advancements in the integration domain of DT and RIS, as well as the exploration and implementation in the UAV systems, as summarized in Table 2.
Reference | System Model | Problem | RIS | DT | UAV | MEC |
[28] | RIS-empowered DT-MEC-URLLC system | Total end-to-end delay minimization problem with URLLC constraints | ||||
[64] | Active RIS-assisted DT-based MEC system | Enhancing performance of low-latency and high-reliability communication services | ||||
[65] | DT-aided and RIS-assisted UCCF system | Maximizing the total rate of the RIS auxiliary uplink UCCF system | ||||
[66] | DTRiD Architecture for 6G IoT Networks | Dynamic resource allocation problem | ||||
[67] | DT-aided edge intelligent network | Insufficient computing power and complex network layout problem | ||||
[68] | Digital Twin-Driven and RIS-aided System | Delay and energy optimization problem | ||||
[69] | A digital twin-based model for the UAV and RIS | Dynamic resource allocation problem | ||||
[70] | RIS-aided multi-tier hybrid computing model for DITEN | Maximization of the total computation rate of the DITEN |
Specifically, authors in [64] propose a scheme integrating active RIS and DT technologies. DT optimizes RIS phase matrices, reducing signal attenuation and interference, while task offloading and edge caching lower energy consumption and latency, enhancing URLLC performance. The scheme accounts for imperfect CSI and employs an alternating optimization algorithm for system optimization. Similarly, the authors in [28] utilize a DT framework to map physical objects and network parameters in real time. The authors propose a DDPG-based algorithm to learn environmental and network states, in order to jointly optimize RIS phase shifts, power and bandwidth allocation, and task offloading. Meanwhile, the study [65] introduces a DT-based learning framework that simulates physical environments, reducing interactions with real environments, lowering system costs, and improving training efficiency. The authors introduce a new position-adaptive binary particle swarm optimization (PABPSO) and two twin delayed deep deterministic policy gradient (TD3) algorithms to optimize user association and power control, maximizing uplink rates in RIS-assisted systems. Similarly, [66] integrates real IoT data into a DT framework, creating diverse datasets to train a DDPG model. The RIS phase shifts are dynamically adjusted based on real-time channel predictions and IoT device locations, optimizing resource allocation and boosting communication efficiency.
Furthermore, authors in [67] propose the DITEN architecture, combining RIS and federated learning. RIS improves channel quality, federated learning enhances model accuracy, and global-local learning strategies accelerate convergence, effectively addressing resource management and task offloading. Similarly, authors in [68] propose a DT-driven and RIS-based task offloading and RIS configuration framework (DTVIF) in the vehicular network scenario. DT monitors IoV environments in real time, while MEC enhances computational capacity and reduces latency and energy consumption. Authors in [69] propose a RIS-assisted system with UAVs as relay nodes. DT technology predicts large-scale fading in future phases, while RIS optimizes the propagation environment in real time to mitigate small-scale fading errors. Authors in [70] present a RIS-assisted multi-level hybrid computing framework for the DITEN system. Over-the-air computation (AirComp) builds the DT layer, while MEC enables precise task offloading under DT’s real-time monitoring. The integration of RIS enhances signal transmission and suppresses interference among heterogeneous nodes, ensuring efficient and reliable communication. Additionally, the authors in [74] also emphasize the critical role of RIS, DT, and UAVs in enhancing network performance through mechanisms such as information fusion, intelligent decision-making, and real-time feedback.
Synthesizing the research above, it becomes evident that the profound integration of RIS and digital twin technology holds immense potential and possibilities within AEC networks. More critically, with the introduction of advanced algorithms such as deep reinforcement learning and transfer learning, the collaborative optimization mechanism between RIS, DT, and UAVs has become more intelligent and efficient. Next, we introduce our proposed MEC network framework that combines RIS, DT, and UAVs.
Through analysis of relevant research, we innovatively propose a UAV-assisted MEC network framework for integrating DT and RIS. The specific architecture is shown in Figure 5. The whole architecture is structured into three layers: the ground layer, the aerial layer, and the digital twin layer. Specifically, the ground layer primarily encompasses mobile devices (MD) and terrestrial RIS (TRIS), where ground devices continually generate computational tasks. The aerial layer comprises UAVs equipped with RIS (ARIS) and UAVs mounted with edge servers (AMEC), which are responsible for the real-time updating of the digital twin layer and undertake the critical task of providing task offloading and computing services for the terminal. Integrating both static and dynamic capabilities of RISs and ARIS, this dual-assistance mechanism significantly optimizes the system’s communication environment.
Moreover, the digital twin layer constructs a detailed environment model, enabling efficient simulation and prediction of strategic solutions. Subsequently, these solutions are transmitted back to the physical layer, offering viable and effective resolutions for dynamic and intricate edge computing network environments. This network framework aims to build an efficient, flexible, scalable AEC system, enabling intelligent management and optimization of complex edge computing environments.
Based on the previous literature review and analysis, we discuss several key issues and challenges for research on the integration of RIS and DT in AEC networks:
Deployment of RIS: The deployment location of RIS is critical to optimizing system performance. To pinpoint the optimal deployment sites for RIS, we must comprehensively consider many critical factors, including the distribution of user density, the characteristics of services required within the target area, and the capability to avoid interference.
Real-time synchronization: DT must accurately reflect the current network state to support up-to-date decision-making methods. Thus, it is critical to ensure real-time synchronization between physical entities and their corresponding digital twins. Any significant update delays or missing data will hinder system performance.
High dynamics of the system: The dynamics of user devices and UAVs pose unprecedented challenges for the phase-shift design of RIS and instantaneous updating of digital twins. Real-time decision-making in this dynamic environment is vital. This necessitates innovative algorithms and technologies to ensure stable, premium services.
In this section, we delve into the innovative research directions of RIS and DT technologies in AEC, with a focus on diversified optimization objectives and the integration of emerging technologies. Our objective is to spark fresh dimensions of thought and technological advancement within this promising field.
In edge computing systems, the issue of energy efficiency consistently holds a paramount position. Numerous studies delve into the problem of enhancing system energy efficiency by harnessing the potential of RIS and digital twin technology. RIS phase shift design optimizes UAV-assisted networks for flexible channel reconstruction, enhancing the system’s energy efficiency [32]. On the other hand, DT can construct a virtual world to optimize the flight trajectories and computation offloading strategies of UAVs [59]. Meanwhile, it dramatically reduces communication overhead during the deep reinforcement learning process.
However, current research on the integrated application of RIS and DT technology remains inadequate. Combining these technologies promises significant system energy efficiency and presents a promising research direction. Specifically, we can leverage RIS to offer more flexible and versatile communication support, minimizing UAVs' inefficient flight paths and harnessing the computational capabilities of edge nodes more efficiently. Simultaneously, with the real-time monitoring capability of digital twin technology, we can accurately grasp the system dynamics to optimize UAV flight trajectories, channel allocation, and RIS phase shift designs. This comprehensive application strategy is expected to provide strong support for the future development of intelligent edge systems.
Currently, research on AEC mainly focuses on UAVs operating at fixed or pre-set altitudes, most of which are single UAV scenarios. Investigations into three-dimensional (3D) trajectory optimization remain understudied [75]. With the emergence of RIS and DT, the flexibility and controllability of UAV flight capabilities significantly expands, facilitating research in this objective.
By deploying aerial RIS with strategic phase shifts, we expand UAVs’ communication coverage while ensuring stability and reliability. Furthermore, we can optimize UAV trajectory plan algorithms and make intelligent decisions by leveraging DT technology’s robust simulation and prediction capabilities. Meanwhile, we can harness distributed execution strategies to enable multiple UAVs to independently and efficiently execute complex 3D trajectories, enhancing their computational capabilities and collaborative operations. In conclusion, integrating RIS and DT promises vast exploration avenues for multi-UAV 3D trajectory design.
In practical application scenarios, the mobility of user devices and UAVs, the randomness of tasks, the blockage of system communication links, and the dynamic variations in channel conditions collectively contribute to a complex and highly dynamic system environment [76]. However, the majority of current research remains grounded in idealized settings, limiting real-world applicability. Fortunately, RIS and DT offer robust solutions for dynamic computation offloading.
RIS dynamically supports the communication environment and facilitates diversified offloading decisions. Meanwhile, DT enables efficient solutions by creating a real-time mapping of the physical world and integrating advanced intelligent algorithms. Consequently, integrating RIS and DT in AEC presents a novel approach to address current challenges and paves the way for future edge intelligence.
Space-air-ground integrated network (SAGIN) represents a multi-dimensional network concept that seamlessly integrates the dimensions, aspiring to forge a comprehensive integrated information network. It not only breaks through the bottleneck of transmission delay caused by the distance of traditional satellite communications but also cleverly compensates for the limitations of UAV communication networks regarding coverage. This holistic approach caters to diverse application demands [77]. However, the SAGIN poses intricate challenges in orchestrating collaboration across different tiers, and its stability may falter in dynamic environments.
To address these practical issues, authors in [78] delve into a SAGIN model, which offers efficient communication and edge computing support tailored for IoT devices in temporary, regional user zones and rescue scenarios. Furthermore, authors in [79] introduce RIS to significantly bolster wireless coverage through dynamic reconfiguration of signal transmission paths in SAGIN. Meanwhile, authors in [80] highlight the potential of DT technology to propel performance enhancements in SAGIN. In conclusion, the profound integration of SAGIN with RIS and DT presents a promising direction for the future of edge computing, ensuring seamless wireless connectivity for users.
Current battery technology limitations hinder UAVs' long-term operations, especially in emergency scenarios. Wireless power transfer (WPT) technologies emerge as innovative solutions to alleviate the energy constraints of the system [81]. The technology can efficiently transfer energy directly to devices such as UAVs by deploying transmitters at the access point, and the feasibility of this approach has been well validated by existing research results [82]. However, WPT faces challenges like short range, complex multi-load management, and sensitivity to misalignment.
To overcome these challenges and optimize the performance of WPT technology, RISs demonstrate remarkable potential in enhancing the transmission efficiency of WPT signals through phase shift adjustments [83]. Additionally, incorporating the monitoring and prediction capabilities of DT technology further strengthens the support provided by WPT technology [84]. By combining these technologies, we can dynamically enhance and optimize the energy replenishment process provided by WPT technology to UAVs, thereby enhancing the overall efficiency of AEC networks.
Federated Learning emerges as a pivotal technology in distributed machine learning, centered on fostering collaborative machine learning among multiple participants without the need for direct data exchange[85]. In this framework, individual agents independently train local models, subsequently aggregating only the crucial weight information of these models at a central coordinator. This unique feature significantly enhances the protection of data privacy and security [86]. Nevertheless, practical applications of federated learning confront challenges, such as the potential for high communication costs in complex network environments and agent selection in heterogeneous environments.
RIS is ingeniously integrated into the federated learning process, effectively shortening training cycles and reducing communication costs [87]. In addition, authors in [88] delve into optimizing the deployment strategies and beamforming techniques of RIS through Federated Learning, aiming to achieve higher user capacity and spectral efficiency. Furthermore, authors in [89] uncover the immense potential of federated learning in DT networks, offering an innovative modeling and data analysis approach. Overall, integrating federated learning, RIS, and DT within AEC networks lays a potential solution for building more innovative, safer, and more efficient future network architectures.
This article presents a comprehensive and insightful overview of the latest research advancements in AEC assisted by DT and RIS. Firstly, we analyze the recent progress of AEC’s research and individually dissect the core elements of RIS and DT. Then, we systematically organize and categorize current research findings to illuminate these technologies' unique value and potential applications. Furthermore, we delve deeply into the possibilities of their integration, showcasing the massive advantage of their collaboration. Through meticulous analysis, we unveil the expansive application prospects of combining DT and RIS with AEC. Looking ahead, the profound integration of DT and RIS in AEC will further enrich and refine the theoretical frameworks of edge computing and intelligent communication, opening up vast horizons and opportunities for future scientific exploration and practical applications.
This work was supported in part by the China Postdoctoral Science Foundation (No. 2024M750199), the National Natural Science Foundation of China (Nos.
[1] |
U. M. Malik, M. A. Javed, S. Zeadally, et al., “Energy-efficient fog computing for 6G-enabled massive IoT: Recent trends and future opportunities,” IEEE Internet of Things Journal, vol. 9, no. 16, pp. 14572–14594, 2021. DOI: 10.1109/JIOT.2021.3068056
|
[2] |
C. W. Luo, J. Zhang, X. L. Cheng, et al., “Computation off-loading in resource-constrained edge computing systems based on deep reinforcement learning,” IEEE Transactions on Computers, vol. 73, no. 1, pp. 109–122, 2024. DOI: 10.1109/TC.2023.3321938
|
[3] |
X. Fan, Y. Wang, Y. Huo, et al., “Efficient distributed swarm learning for edge computing,” in Proceedings of the ICC 2023-IEEE International Conference on Communications, Rome, Italy, pp. 3627–3632, 2023.
|
[4] |
J. W. Huang, F. Z. Liu, and J. B. Zhang, “Multi-dimensional QoS evaluation and optimization of mobile edge computing for IoT: A survey,” Chinese Journal of Electronics, vol. 33, no. 4, pp. 859–874, 2024. DOI: 10.23919/cje.2023.00.264
|
[5] |
Y. L. Wu, P. Tian, Y. W. Cao, et al., “Edge computing-based mobile object tracking in internet of things,” High-Confidence Computing, vol. 2, no. 1, article no. 100045, 2022. DOI: 10.1016/j.hcc.2021.100045
|
[6] |
J. M. Xia, Y. F. Liu, and L. Tan, “Joint optimization of trajectory and task offloading for cellular-connected multi-UAV mobile edge computing,” Chinese Journal of Electronics, vol. 33, no. 3, pp. 823–832, 2024. DOI: 10.23919/cje.2022.00.159
|
[7] |
F. Pervez, A. Sultana, C. G. Yang, et al., “Energy and latency efficient joint communication and computation optimization in a multi-UAV-assisted MEC network,” IEEE Transactions on Wireless Communications, vol. 23, no. 3, pp. 1728–1741, 2024. DOI: 10.1109/TWC.2023.3291692
|
[8] |
Y. B. Qu, Z. H. Wei, Z. Qin, et al., “Collaborative service provisioning for UAV-assisted mobile edge computing,” Chinese Journal of Electronics, vol. 33, no. 6, pp. 1504–1514, 2024. DOI: 10.23919/cje.2021.00.323
|
[9] |
Q. L. Zhang, Y. Luo, H. Jiang, et al., “Aerial edge computing: A survey,” IEEE Internet of Things Journal, vol. 10, no. 16, pp. 14357–14374, 2023. DOI: 10.1109/JIOT.2023.3263360
|
[10] |
Z. Y. Song, X. T. Qin, Y. Y. Hao, et al., “A comprehensive survey on aerial mobile edge computing: Challenges, state-of-the-art, and future directions,” Computer Communications, vol. 191, pp. 233–256, 2022. DOI: 10.1016/j.comcom.2022.05.004
|
[11] |
S. H. Alsamhi, A. V. Shvetsov, S. Kumar, et al., “Computing in the sky: A survey on intelligent ubiquitous computing for UAV-assisted 6g networks and industry 4.0/5.0,” Drones, vol. 6, no. 7, article no. 177, 2022. DOI: 10.3390/drones6070177
|
[12] |
A. C. Pogaku, D. T. Do, B. M. Lee, et al., “UAV-assisted RIS for future wireless communications: A survey on optimization and performance analysis,” IEEE Access, vol. 10, pp. 16320–16336, 2022. DOI: 10.1109/ACCESS.2022.3149054
|
[13] |
X. W. Pang, M. Sheng, N. Zhao, et al., “When UAV meets IRS: Expanding air-ground networks via passive reflection,” IEEE Wireless Communications, vol. 28, no. 5, pp. 164–170, 2021. DOI: 10.1109/MWC.010.2000528
|
[14] |
B. D. Shang, H. V. Poor, and L. J. Liu, “Aerial reconfigurable intelligent surfaces meet mobile edge computing,” IEEE Wireless Communications, vol. 29, no. 6, pp. 104–111, 2022. DOI: 10.1109/MWC.001.2200009
|
[15] |
Y. W. Wu, K. Zhang, and Y. Zhang, “Digital twin networks: A survey,” IEEE Internet of Things Journal, vol. 8, no. 18, pp. 13789–13804, 2021. DOI: 10.1109/JIOT.2021.3079510
|
[16] |
F. X. Tang, X. H. Chen, T. K. Rodrigues, et al., “Survey on digital twin edge networks (DITEN) toward 6G,” IEEE Open Journal of the Communications Society, vol. 3, pp. 1360–1381, 2022. DOI: 10.1109/OJCOMS.2022.3197811
|
[17] |
Y. B. Xie, L. Shi, Z. C. Wei, et al., “An energy-efficient resource allocation strategy in massive MIMO-enabled vehicular edge computing networks,” High-Confidence Computing, vol. 3, no. 3, article no. 100130, 2023. DOI: 10.1016/j.hcc.2023.100130
|
[18] |
Y. X. He, D. S. Zhai, R. N. Zhang, et al., “A mobile edge computing framework for task offloading and resource allocation in UAV-assisted VANETs,” in IEEE INFOCOM 2021-IEEE Conference on Computer Communications Workshops (INFOCOM WKSHPS), Vancouver, BC, Canada, pp. 1–6, 2021.
|
[19] |
M. Yan, R. Xiong, Y. Wang, et al., “Edge computing task offloading optimization for a UAV-assisted internet of vehicles via deep reinforcement learning,” IEEE Transactions on Vehicular Technology, vol. 73, no. 4, pp. 5647–5658, 2024. DOI: 10.1109/TVT.2023.3331363
|
[20] |
X. X. Dai, Z. Xiao, H. B. Jiang, et al., “UAV-assisted task offloading in vehicular edge computing networks,” IEEE Transactions on Mobile Computing, vol. 23, no. 4, pp. 2520–2534, 2024. DOI: 10.1109/TMC.2023.3259394
|
[21] |
H. Ijaz, R. Ahmad, R. Ahmed, et al., “A UAV-assisted edge framework for real-time disaster management,” IEEE Transactions on Geoscience and Remote Sensing, vol. 61, article no. 1001013, 2023. DOI: 10.1109/TGRS.2023.3306151
|
[22] |
Z. Shah, U. Javed, M. Naeem, et al., “Mobile edge computing (MEC)-enabled UAV placement and computation efficiency maximization in disaster scenario,” IEEE Transactions on Vehicular Technology, vol. 72, no. 10, pp. 13406–13416, 2023. DOI: 10.1109/TVT.2023.3274107
|
[23] |
G. Sun, L. He, Z. M. Sun, et al., “Joint task offloading and resource allocation in aerial-terrestrial UAV networks with edge and fog computing for post-disaster rescue,” IEEE Transactions on Mobile Computing, vol. 23, no. 6, pp. 8582–8600, 2024. DOI: 10.1109/TMC.2024.3350886
|
[24] |
Y. Z. Liao, L. Liu, Y. Y. Song, et al., “Joint communication-caching-computing resource allocation for bidirectional data computation in IRS-assisted hybrid UAV-terrestrial network,” Chinese Journal of Electronics, vol. 33, no. 4, pp. 1093–1103, 2024. DOI: 10.23919/cje.2023.00.089
|
[25] |
T. Bai, C. H. Pan, Y. S. Deng, et al., “Latency minimization for intelligent reflecting surface aided mobile edge computing,” IEEE Journal on Selected Areas in Communications, vol. 38, no. 11, pp. 2666–2682, 2020. DOI: 10.1109/JSAC.2020.3007035
|
[26] |
Z. Mohamed and S. Aïssa, “Leveraging UAVs with intelligent reflecting surfaces for energy-efficient communications with cell-edge users,” in Proceedings of the 2020 IEEE International Conference on Communications Workshops (ICC Workshops), Dublin, Ireland, pp. 1–6, 2020.
|
[27] |
H. Z. Guo, X. Y. Zhou, J. D. Wang, et al., “Intelligent task offloading and resource allocation in digital twin based aerial computing networks,” IEEE Journal on Selected Areas in Communications, vol. 41, no. 10, pp. 3095–3110, 2023. DOI: 10.1109/JSAC.2023.3310067
|
[28] |
S. Kurma, M. Katwe, K. Singh, et al., “RIS-empowered MEC for URLLC systems with digital-twin-driven architecture,” IEEE Transactions on Communications, vol. 72, no. 4, pp. 1983–1997, 2024. DOI: 10.1109/TCOMM.2023.3333345
|
[29] |
S. A. H. Mohsan, M. A. Khan, M. H. Alsharif, et al., “Intelligent reflecting surfaces assisted UAV communications for massive networks: Current trends, challenges, and research directions,” Sensors, vol. 22, no. 14, article no. 5278, 2022. DOI: 10.3390/s22145278
|
[30] |
M. Ahmed, A. Wahid, S. S. Laique, et al., “A survey on STAR-RIS: Use cases, recent advances, and future research challenges,” IEEE Internet of Things Journal, vol. 10, no. 16, pp. 14689–14711, 2023. DOI: 10.1109/JIOT.2023.3279357
|
[31] |
Q. L. Liu, J. Han, and Q. Liu, “Joint task offloading and resource allocation for RIS-assisted UAV for mobile edge computing networks,” in Proceedings of the 2023 IEEE/CIC International Conference on Communications in China (ICCC), Dalian, China, pp. 1–6, 2023.
|
[32] |
E. T. Michailidis, N. I. Miridakis, A. Michalas, et al., “Energy optimization in dual-RIS UAV-aided MEC-enabled internet of vehicles,” Sensors, vol. 21, no. 13, article no. 4392, 2021. DOI: 10.3390/s21134392
|
[33] |
X. T. Qin, Z. Y. Song, T. W. Hou, et al., “Joint optimization of resource allocation, phase shift, and UAV trajectory for energy-efficient RIS-assisted UAV-enabled MEC systems,” IEEE Transactions on Green Communications and Networking, vol. 7, no. 4, pp. 1778–1792, 2023. DOI: 10.1109/TGCN.2023.3287604
|
[34] |
Z. Y. Zhai, X. H. Dai, B. Duo, et al., “Energy-efficient UAV-mounted RIS assisted mobile edge computing,” IEEE Wireless Communications Letters, vol. 11, no. 12, pp. 2507–2511, 2022. DOI: 10.1109/LWC.2022.3206587
|
[35] |
Q. S. Ai, X. H. Qiao, Y. Z. Liao, et al., “Joint optimization of USVs communication and computation resource in IRS-aided wireless inland ship MEC networks,” IEEE Transactions on Green Communications and Networking, vol. 6, no. 2, pp. 1023–1036, 2022. DOI: 10.1109/TGCN.2021.3135530
|
[36] |
Y. N. Shnaiwer and M. Kaneko, “Minimizing IoT energy consumption by IRS-aided UAV mobile edge computing,” IEEE Networking Letters, vol. 5, no. 1, pp. 16–20, 2023. DOI: 10.1109/LNET.2022.3222452
|
[37] |
B. Duo, M. L. He, Q. Q. Wu, et al., “Joint dual-UAV trajectory and RIS design for ARIS-assisted aerial computing in IoT,” IEEE Internet of Things Journal, vol. 10, no. 22, pp. 19584–19594, 2023. DOI: 10.1109/JIOT.2023.3288213
|
[38] |
L. P. Li, W. Q. Guan, C. Zhao, et al., “Trajectory planning, phase shift design, and IoT devices association in flying-RIS-assisted mobile edge computing,” IEEE Internet of Things Journal, vol. 11, no. 1, pp. 147–157, 2024. DOI: 10.1109/JIOT.2023.3300700
|
[39] |
M. Asim, M. ELAffendi, and A. A. Abd El-Latif, “Multi-IRS and multi-UAV-assisted MEC system for 5G/6G networks: Efficient joint trajectory optimization and passive beamforming framework,” IEEE Transactions on Intelligent Transportation Systems, vol. 24, no. 4, pp. 4553–4564, 2023. DOI: 10.1109/TITS.2022.3178896
|
[40] |
S. H. Liu, Y. C. Huang, H. Hu, et al., “Resource and trajectory optimization for secure communication in RIS assisted UAV-MEC system,” IET Communications, vol. 17, no. 18, pp. 2068–2079, 2023. DOI: 10.1049/cmu2.12680
|
[41] |
M. Hadi and R. Ghazizadeh, “Computation bits enhancement for IRS-assisted multi-UAV wireless powered mobile edge computing systems,” Vehicular Communications, vol. 43, article no. 100656, 2023. DOI: 10.1016/j.vehcom.2023.100656
|
[42] |
Y. Xu, T. K. Zhang, Y. X. Zou, et al., “Reconfigurable intelligence surface aided UAV-MEC systems with NOMA,” IEEE Communications Letters, vol. 26, no. 9, pp. 2121–2125, 2022. DOI: 10.1109/LCOMM.2022.3183285
|
[43] |
A. V. Savkin, C. Huang, and W. Ni, “Joint multi-UAV path planning and LoS communication for mobile-edge computing in IoT networks with RISs,” IEEE Internet of Things Journal, vol. 10, no. 3, pp. 2720–2727, 2023. DOI: 10.1109/JIOT.2022.3215255
|
[44] |
X. Fan, Y. Wang, Y. Huo, et al., “BEV-SGD: Best effort voting SGD against byzantine attacks for analog-aggregation-based federated learning over the air,” IEEE Internet of Things Journal, vol. 9, no. 19, pp. 18946–18959, 2022. DOI: 10.1109/JIOT.2022.3164339
|
[45] |
X. Fan, Y. Wang, Y. Huo, et al., “Joint optimization for federated learning over the air,” in Proceedings of the ICC 2022-IEEE International Conference on Communications, Seoul, Korea, pp. 2798–2803, 2022.
|
[46] |
Q. Q. Wu and R. Zhang, “Intelligent reflecting surface enhanced wireless network via joint active and passive beamforming,” IEEE Transactions on Wireless Communications, vol. 18, no. 11, pp. 5394–5409, 2019. DOI: 10.1109/TWC.2019.2936025
|
[47] |
A. Mahmood, T. X. Vu, W. U. Khan, et al., “Optimizing computational and communication resources for MEC network empowered UAV-RIS communication,” in Proceedings of the 2022 IEEE Globecom Workshops (GC Wkshps), Rio de Janeiro, Brazil, pp. 974–979, 2022.
|
[48] |
B. D. Shang, R. Shafin, and L. J. Liu, “UAV swarm-enabled aerial reconfigurable intelligent surface (SARIS),” IEEE Wireless Communications, vol. 28, no. 5, pp. 156–163, 2021. DOI: 10.1109/MWC.010.2000526
|
[49] |
T. Liu, L. Tang, W. L. Wang, et al., “Digital-twin-assisted task offloading based on edge collaboration in the digital twin edge network,” IEEE Internet of Things Journal, vol. 9, no. 2, pp. 1427–1444, 2022. DOI: 10.1109/JIOT.2021.3086961
|
[50] |
S. Shen, Y. J. Fu, and K. Yang, “Joint resource allocation in digital-twin-assisted and UAV-enabled mobile edge computing system,” in Proceedings of the 2023 IEEE 3rd International Conference on Electronic Technology, Communication and Information (ICETCI), Changchun, China, pp. 302–306, 2023.
|
[51] |
Y. J. Li, D. Van Huynh, T. Do-Duy, et al., “Unmanned aerial vehicle-aided edge networks with ultra-reliable low-latency communications: A digital twin approach,” IET Signal Processing, vol. 16, no. 8, pp. 897–908, 2022. DOI: 10.1049/sil2.12128
|
[52] |
B. Hazarika, K. Singh, C. P. Li, et al., “RADiT: Resource allocation in digital twin-driven UAV-aided internet of vehicle networks,” IEEE Journal on Selected Areas in Communications, vol. 41, no. 11, pp. 3369–3385, 2023. DOI: 10.1109/JSAC.2023.3310048
|
[53] |
A. Paul, K. Singh, M. H. T. Nguyen, et al., “Digital twin-assisted space-air-ground integrated networks for vehicular edge computing,” IEEE Journal of Selected Topics in Signal Processing, vol. 18, no. 1, pp. 66–82, 2024. DOI: 10.1109/JSTSP.2023.3340107
|
[54] |
Q. Guo, F. X. Tang, and N. Kato, “Resource allocation for aerial assisted digital twin edge mobile network,” IEEE Journal on Selected Areas in Communications, vol. 41, no. 10, pp. 3070–3079, 2023. DOI: 10.1109/JSAC.2023.3310065
|
[55] |
W. Sun, P. Wang, N. Xu, et al., “Dynamic digital twin and distributed incentives for resource allocation in aerial-assisted internet of vehicles,” IEEE Internet of Things Journal, vol. 9, no. 8, pp. 5839–5852, 2022. DOI: 10.1109/JIOT.2021.3058213
|
[56] |
G. Q. Wu, J. T. Nie, J. H. Tang, et al., “UAV-assisted data synchronization for digital-twin-enabled vehicular networks,” in Proceedings of the 2023 IEEE/CIC International Conference on Communications in China (ICCC), Dalian, China, pp. 1–6, 2023.
|
[57] |
N. T. Hoa, L. Van Huy, B. D. Son, et al., “Dynamic offloading for edge computing-assisted metaverse systems,” IEEE Communications Letters, vol. 27, no. 7, pp. 1749–1753, 2023. DOI: 10.1109/LCOMM.2023.3274649
|
[58] |
J. L. Shi, C. Y. Li, Y. C. Guan, et al., “Multi-UAV-assisted computation offloading in DT-based networks: A distributed deep reinforcement learning approach,” Computer Communications, vol. 210, pp. 217–228, 2023. DOI: 10.1016/j.comcom.2023.07.041
|
[59] |
B. Li, Y. F. Liu, L. Tan, et al., “Digital twin assisted task offloading for aerial edge computing and networks,” IEEE Transactions on Vehicular Technology, vol. 71, no. 10, pp. 10863–10877, 2022. DOI: 10.1109/TVT.2022.3182647
|
[60] |
L. Zhao, S. Li, Y. C. Guan, et al., “Adaptive multi-UAV trajectory planning leveraging digital twin technology for urban IIoT applications,” IEEE Transactions on Network Science and Engineering, vol. 11, no. 6, pp. 5349–5363, 2024. DOI: 10.1109/TNSE.2023.3344428
|
[61] |
K. Xiong, Z. H. Wang, S. P. Leng, et al., “A digital-twin-empowered lightweight model-sharing scheme for multirobot systems,” IEEE Internet of Things Journal, vol. 10, no. 19, pp. 17231–17242, 2023. DOI: 10.1109/JIOT.2023.3273402
|
[62] |
W. S. Liu, B. Li, W. C. Xie, et al., “Energy efficient computation offloading in aerial edge networks with multi-agent cooperation,” IEEE Transactions on Wireless Communications, vol. 22, no. 9, pp. 5725–5739, 2023. DOI: 10.1109/TWC.2023.3235997
|
[63] |
X. Fan, Y. Wang, Y. Huo, et al., “Best effort voting power control for byzantine-resilient federated learning over the air,” in Proceedings of the 2022 IEEE International Conference on Communications Workshops (ICC Workshops), Seoul, Korea, pp. 806–811, 2022.
|
[64] |
T. A. Lestari, S. Kurma, K. Singh, et al., “Active-RIS-assisted digital twin-based URLLC internet-of-things networks,” in Proceedings of the GLOBECOM 2023-2023 IEEE Global Communications Conference, Kuala Lumpur, Malaysia, pp. 7079–7084, 2023.
|
[65] |
Y. P. Cui, T. J. Lv, W. Ni, et al., “Digital twin-aided learning for managing reconfigurable intelligent surface-assisted, uplink, user-centric cell-free systems,” IEEE Journal on Selected Areas in Communications, vol. 41, no. 10, pp. 3175–3190, 2023. DOI: 10.1109/JSAC.2023.3310050
|
[66] |
M. Tariq, S. Ahmad, and H. V. Poor, “Dynamic resource allocation in IoT enhanced by digital twins and intelligent reflecting surfaces,” IEEE Internet of Things Journal, vol. 11, no. 16, pp. 27295–27302, 2024. DOI: 10.1109/JIOT.2024.3398413
|
[67] |
Y. Y. Dai, J. Wu, J. T. Zhao, et al., “Intelligent reflecting surfaces aided task offloading in digital twin edge networks,” in Proceedings of the 2023 IEEE 98th Vehicular Technology Conference (VTC2023-Fall), Hong Kong, China, pp. 1–5, 2023.
|
[68] |
X. M. Yuan, J. H. Chen, N. Zhang, et al., “Digital twin-driven vehicular task offloading and IRS configuration in the internet of vehicles,” IEEE Transactions on Intelligent Transportation Systems, vol. 23, no. 12, pp. 24290–24304, 2022. DOI: 10.1109/TITS.2022.3204585
|
[69] |
M. M. Wu, Y. Xiao, Y. L. Gao, et al., “Digital twin for UAV-RIS assisted vehicular communication systems,” IEEE Transactions on Wireless Communications, vol. 23, no. 7, pp. 7638–7651, 2024. DOI: 10.1109/TWC.2023.3342991
|
[70] |
Y. P. Zhao, Q. Q. Wu, G. J. Chen, et al., “Intelligent reflecting surface aided multi-tier hybrid computing,” IEEE Journal of Selected Topics in Signal Processing, vol. 18, no. 1, pp. 83–97, 2024. DOI: 10.1109/JSTSP.2023.3332455
|
[71] |
A. Konikov and L. Surkova, “Monitoring of the transport movement zone using UAV and geostationary satellite,” Transportation Research Procedia, vol. 63, pp. 1589–1594, 2022. DOI: 10.1016/j.trpro.2022.06.172
|
[72] |
A. Broekman, P. J. Gräbe, and W. J. Steyn, “Real-time traffic quantization using a mini edge artificial intelligence platform,” Transportation Engineering, vol. 4, article no. 100068, 2021. DOI: 10.1016/j.treng.2021.100068
|
[73] |
F. Edemetti, A. Maiale, C. Carlini, et al., “Vineyard digital twin: Construction and characterization via UAV images–DIWINE proof of concept,” in Proceedings of the 2022 IEEE 23rd International Symposium on A World of Wireless, Mobile and Multimedia Networks (WoWMoM), Belfast, United Kingdom, pp. 601–606, 2022.
|
[74] |
A. Masaracchia, D. Van Huynh, G. C. Alexandropoulos, et al., “Toward the metaverse realization in 6G: Orchestration of RIS-enabled smart wireless environments via digital twins,” IEEE Internet of Things Magazine, vol. 7, no. 2, pp. 22–28, 2024. DOI: 10.1109/IOTM.001.2300128
|
[75] |
F. Z. Zeng, Z. Z. Hu, Z. Xiao, et al., “Resource allocation and trajectory optimization for QoE provisioning in energy-efficient UAV-enabled wireless networks,” IEEE Transactions on Vehicular Technology, vol. 69, no. 7, pp. 7634–7647, 2020. DOI: 10.1109/TVT.2020.2986776
|
[76] |
S. C. Zhu, L. Gui, D. M. Zhao, et al., “Learning-based computation offloading approaches in UAVs-assisted edge computing,” IEEE Transactions on Vehicular Technology, vol. 70, no. 1, pp. 928–944, 2021. DOI: 10.1109/TVT.2020.3048938
|
[77] |
M. Jia, J. Wu, L. Zhang, et al., “Joint optimization communication and computing resource for LEO satellites with edge computing,” Chinese Journal of Electronics, vol. 32, no. 5, pp. 1011–1021, 2023. DOI: 10.23919/cje.2022.00.314
|
[78] |
Z. Z. Hu, F. Z. Zeng, Z. Xiao, et al., “Joint resources allocation and 3D trajectory optimization for UAV-enabled space-air-ground integrated networks,” IEEE Transactions on Vehicular Technology, vol. 72, no. 11, pp. 14214–14229, 2023. DOI: 10.1109/TVT.2023.3280482
|
[79] |
X. L. Cao, B. Yang, C. W. Huang, et al., “Converged reconfigurable intelligent surface and mobile edge computing for space information networks,” IEEE Network, vol. 35, no. 4, pp. 42–48, 2021. DOI: 10.1109/MNET.011.2100049
|
[80] |
Q. Chen, Z. Guo, W. X. Meng, et al., “A survey on resource management in joint communication and computing-embedded SAGIN,” IEEE Communications Surveys & Tutorials, in press, 2024.
|
[81] |
J. Zhang, C. W. Luo, N. Liu, et al., “Minimizing charging task time of WRSN assisted with multiple MUVs and laser-charged UAVs,” High-Confidence Computing, vol. 5, no. 2, article no. 100272, 2025. DOI: 10.1016/j.hcc.2024.100272
|
[82] |
S. Lee, N. Lim, W. Choi, et al., “Study on battery charging converter for MPPT control of laser wireless power transmission system,” Electronics, vol. 9, no. 10, article no. 1745, 2020. DOI: 10.3390/electronics9101745
|
[83] |
G. Li, M. Zeng, D. Mishra, et al., “Latency minimization for IRS-aided NOMA MEC systems with WPT-enabled IoT devices,” IEEE Internet of Things Journal, vol. 10, no. 14, pp. 12156–12168, 2023. DOI: 10.1109/JIOT.2023.3240395
|
[84] |
A. Taneja and S. Rani, “Digital twin empowered approach for sustainable IoT in consumer electronics health: A use case,” IEEE Transactions on Consumer Electronics, vol. 70, no. 3, pp. 5667–5674, 2024. DOI: 10.1109/TCE.2024.3417524
|
[85] |
X. Fan, Y. Wang, Y. Huo, et al., “Joint optimization of communications and federated learning over the air,” IEEE Transactions on Wireless Communications, vol. 21, no. 6, pp. 4434–4449, 2022. DOI: 10.1109/TWC.2021.3130111
|
[86] |
X. Fan, Y. Wang, Y. Huo, et al., “1-bit compressive sensing for efficient federated learning over the air,” IEEE Transactions on Wireless Communications, vol. 22, no. 3, pp. 2139–2155, 2023. DOI: 10.1109/TWC.2022.3209190
|
[87] |
W. L. Ni, Y. W. Liu, Z. H. Yang, et al., “Federated learning in multi-RIS-aided systems,” IEEE Internet of Things Journal, vol. 9, no. 12, pp. 9608–9624, 2022. DOI: 10.1109/JIOT.2021.3130444
|
[88] |
R. K. Zhong, X. Liu, Y. W. Liu, et al., “Mobile reconfigurable intelligent surfaces for NOMA networks: Federated learning approaches,” IEEE Transactions on Wireless Communications, vol. 21, no. 11, pp. 10020–10034, 2022. DOI: 10.1109/TWC.2022.3181747
|
[89] |
L. U. Khan, E. Mustafa, J. Shuja, et al., “Federated learning for digital twin-based vehicular networks: Architecture and challenges,” IEEE Wireless Communications, vol. 31, no. 2, pp. 156–162, 2024. DOI: 10.1109/MWC.012.2200373
|
Reference | Contribution | RIS | DT | UAV | MEC |
[9] | Review on the architecture, challenges, performance metrics, and applied state-of-the-art technologies for aerial edge computing | ||||
[10] | Survey on the benefits, challenges, and research advances in UAV-enabled edge computing | ||||
[11] | Comprehensive review of the use of UAVs in 6G networks, emphasizing their utility in mission offloading and data latency challenges | ||||
[12] | Survey on the advantages and potential applications of RIS in UAV support systems | ||||
[13] | Survey on the opportunities and related challenges of integrating UAV and RIS technologies for enhancing air-ground network performance | ||||
[14] | Review on how aerial RIS improves the performance of mobile edge computing networks and discusses challenges and opportunities | ||||
[15] | Review on the main features, key technologies, challenges, application scenarios and new research dynamics of digital twin networks | ||||
[16] | Survey on the application, design, typical scenarios, and challenges faced by digital twin edge networks in 6G | ||||
This paper | Survey on the advancements, challenges, potential, and new opportunities of RIS and digital twin technologies in UAV-assisted edge computing systems |
Reference | System Model | Problem | RIS | DT | UAV | MEC |
[28] | RIS-empowered DT-MEC-URLLC system | Total end-to-end delay minimization problem with URLLC constraints | ||||
[64] | Active RIS-assisted DT-based MEC system | Enhancing performance of low-latency and high-reliability communication services | ||||
[65] | DT-aided and RIS-assisted UCCF system | Maximizing the total rate of the RIS auxiliary uplink UCCF system | ||||
[66] | DTRiD Architecture for 6G IoT Networks | Dynamic resource allocation problem | ||||
[67] | DT-aided edge intelligent network | Insufficient computing power and complex network layout problem | ||||
[68] | Digital Twin-Driven and RIS-aided System | Delay and energy optimization problem | ||||
[69] | A digital twin-based model for the UAV and RIS | Dynamic resource allocation problem | ||||
[70] | RIS-aided multi-tier hybrid computing model for DITEN | Maximization of the total computation rate of the DITEN |